CT image super-resolution reconstruction method based on generative adversarial network
A super-resolution reconstruction and CT image technology, which is applied in biological neural network models, image data processing, neural learning methods, etc., can solve problems affecting doctors' accurate diagnosis, CT image quality degradation, and reduction of projection data, etc.
- Summary
- Abstract
- Description
- Claims
- Application Information
AI Technical Summary
Problems solved by technology
Method used
Image
Examples
Embodiment Construction
[0067] In order to make the technical problems, technical solutions and beneficial effects to be solved by the present invention clearer, the present invention will be further described in detail below in conjunction with the accompanying drawings and embodiments. It should be understood that the specific embodiments described here are only used to explain the present invention, not to limit the present invention.
[0068] Based on the CT image super-resolution reconstruction method based on generative confrontation network, a new generator structure is proposed and a new cost function is designed to reconstruct the CT image by 4 times from 128*128 to 512*512.
[0069] 1. Arrange a multi-layer dense residual block (Multiple Dense Residual Blocks, MDRBs) generator network:
[0070] This application proposes a lightweight multi-level dense residual block structure as the main structure of the generator, such as figure 1 As shown, n residual elements are bundled into a residual ...
PUM
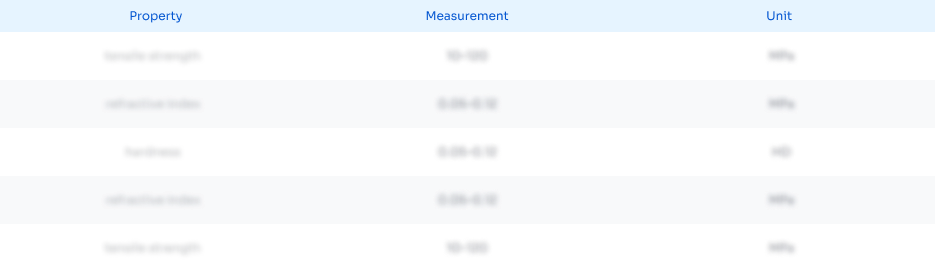
Abstract
Description
Claims
Application Information
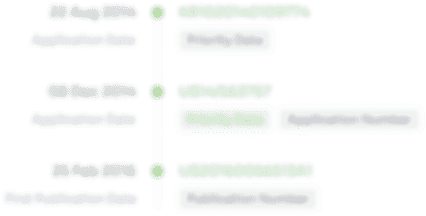
- Generate Ideas
- Intellectual Property
- Life Sciences
- Materials
- Tech Scout
- Unparalleled Data Quality
- Higher Quality Content
- 60% Fewer Hallucinations
Browse by: Latest US Patents, China's latest patents, Technical Efficacy Thesaurus, Application Domain, Technology Topic, Popular Technical Reports.
© 2025 PatSnap. All rights reserved.Legal|Privacy policy|Modern Slavery Act Transparency Statement|Sitemap|About US| Contact US: help@patsnap.com