An Indoor Localization Method Based on Depth Migration and Model Parameter Integration
A technology of model parameters and indoor positioning, which is applied in neural learning methods, character and pattern recognition, biological neural network models, etc. It can solve the problems of reduced distribution differences, insufficient stability and insufficient output, and achieves reduced domain differences and positioning High precision and stable result output
- Summary
- Abstract
- Description
- Claims
- Application Information
AI Technical Summary
Problems solved by technology
Method used
Image
Examples
Embodiment
[0096] This model is used to experiment with the RSS public data set collected at Jaume I University in Spain. The area of the data collection area is about 308.4 square meters, which is divided into 48 grid points, covering a total of 620 access points. Use the samples and labels collected in the first month as fixed source domain data, including a total of 8640 samples; use the target domain data in the nth month (n≥2) as auxiliary training data, and the number of samples is 3120; use the first month Each piece of RSS data received in real time in (n+1) months is used as test data to verify the effect of the model.
[0097] The number of neurons in each layer of the neural network is 256, 256, 256, 256 and 10 in turn, and the initialization parameters are set to random initialization.
[0098] The present invention designs three groups of experiments to verify the superiority of the proposed algorithm. The first group of experiments is to compare the positioning cumulativ...
PUM
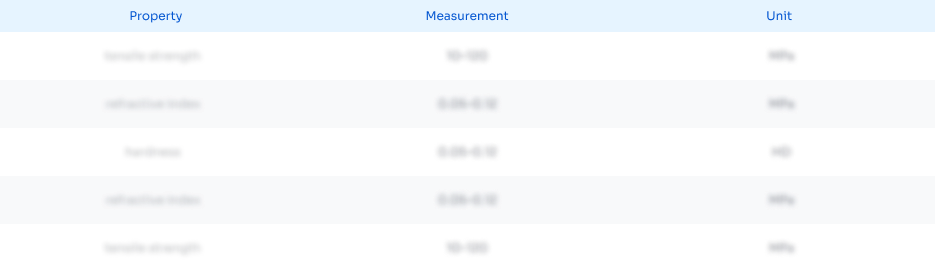
Abstract
Description
Claims
Application Information
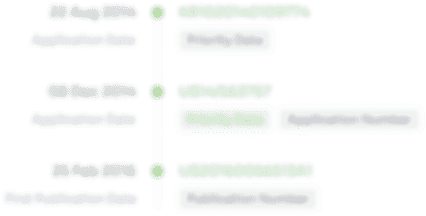
- Generate Ideas
- Intellectual Property
- Life Sciences
- Materials
- Tech Scout
- Unparalleled Data Quality
- Higher Quality Content
- 60% Fewer Hallucinations
Browse by: Latest US Patents, China's latest patents, Technical Efficacy Thesaurus, Application Domain, Technology Topic, Popular Technical Reports.
© 2025 PatSnap. All rights reserved.Legal|Privacy policy|Modern Slavery Act Transparency Statement|Sitemap|About US| Contact US: help@patsnap.com