Method for segmenting capillaries in microscopic image based on generative adversarial network
A microscopic image, generative technology, applied in image analysis, biological neural network model, image enhancement, etc., can solve problems such as low contrast, broken blood vessels, redundant details of blood vessel branches, etc., to remove noise, accelerate training, and enhance The effect of discriminative ability
- Summary
- Abstract
- Description
- Claims
- Application Information
AI Technical Summary
Problems solved by technology
Method used
Image
Examples
specific Embodiment approach 1
[0040] Specific Embodiments 1. A method for segmenting microvessels in a microscopic image based on a generative confrontation network described in this embodiment, the method includes the following steps:
[0041] Step 1: Establish a training model and sample set based on a generative confrontation network;
[0042] Step 2: Input the color fundus image in the sample set into the generation model, extract the image feature information and output the microvascular probability image under the microscopic image as the generation sample;
[0043] Step 3: Use RGB three-channel microscopic images for network training, and perform enhanced processing on microscopic image contrast adaptive histogram equalization;
[0044] Step 4: Increase the amount of training data to re-enhance the microscopic image processed by the preprocessing unit;
[0045]Step 5: Input the generated sample and the corresponding real sample into the discriminant model at the same time, and the discriminant mode...
specific Embodiment approach 2
[0051] Specific Embodiment 2. This embodiment is a further description of the microvascular segmentation method in microscopic images based on the generative confrontation network described in the specific embodiment 1. The generative network model includes a contraction path, an expansion path, and an output layer.
specific Embodiment approach 3
[0052] Specific Embodiment 3. This embodiment is a further description of the microvascular segmentation method in microscopic images based on Generative Adversarial Network described in Embodiment 1. The shrinkage path mainly consists of multiple resnet convolution blocks and Downsampling is composed, and the feature extraction part of the contraction path adopts the idea of resnet network.
PUM
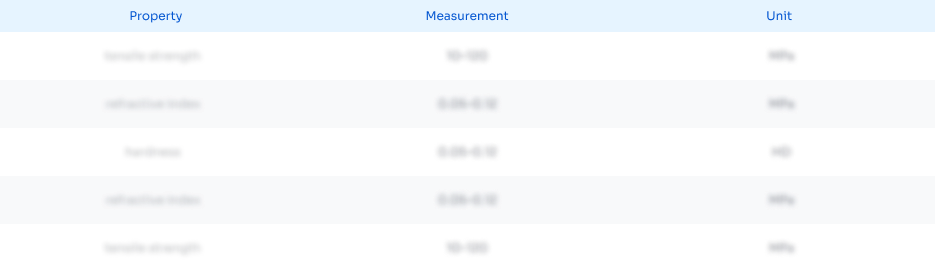
Abstract
Description
Claims
Application Information
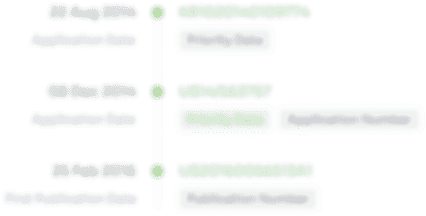
- R&D Engineer
- R&D Manager
- IP Professional
- Industry Leading Data Capabilities
- Powerful AI technology
- Patent DNA Extraction
Browse by: Latest US Patents, China's latest patents, Technical Efficacy Thesaurus, Application Domain, Technology Topic.
© 2024 PatSnap. All rights reserved.Legal|Privacy policy|Modern Slavery Act Transparency Statement|Sitemap