Semantic segmentation method in automatic driving scene based on BiSeNet
A technology for semantic segmentation and automatic driving, applied in combustion engines, internal combustion piston engines, instruments, etc., can solve problems such as time-consuming, and achieve the effect of small model size, high accuracy and good convergence.
- Summary
- Abstract
- Description
- Claims
- Application Information
AI Technical Summary
Problems solved by technology
Method used
Image
Examples
Embodiment Construction
[0046] The present invention will be further described below in conjunction with the accompanying drawings and embodiments.
[0047] Please refer to figure 1 , the present invention provides a semantic segmentation method based on a BiSeNet-based automatic driving scene, comprising the following steps:
[0048] Step S1: collecting urban street image data and preprocessing;
[0049] Step S2: labeling the preprocessed image data to obtain the labeled image data;
[0050] Step S3: performing data enhancement on the labeled image data, and using the enhanced image data as a training set;
[0051] Step S4: Construct the BiSeNet neural network model, and train the model based on the training set;
[0052] Step S5: Preprocess the video information collected by the camera, and perform semantic segmentation on the city streets in the camera according to the trained BiSeNet neural network model.
[0053] Further, the step S1 is specifically:
[0054] Step S11: analyze the categorie...
PUM
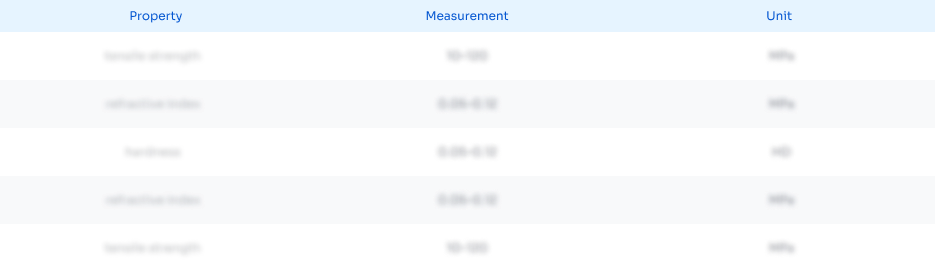
Abstract
Description
Claims
Application Information
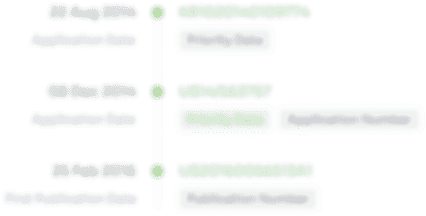
- R&D Engineer
- R&D Manager
- IP Professional
- Industry Leading Data Capabilities
- Powerful AI technology
- Patent DNA Extraction
Browse by: Latest US Patents, China's latest patents, Technical Efficacy Thesaurus, Application Domain, Technology Topic, Popular Technical Reports.
© 2024 PatSnap. All rights reserved.Legal|Privacy policy|Modern Slavery Act Transparency Statement|Sitemap|About US| Contact US: help@patsnap.com