Planning sub-target merging method based on deep learning
A deep learning and sub-goal technology, applied in the field of deep learning-based planning sub-goal merging, can solve problems such as abnormal search method, reduced solution ability, wrong sorting, etc., achieve a balance between solution quality and solution efficiency, and reduce incompleteness Effect
- Summary
- Abstract
- Description
- Claims
- Application Information
AI Technical Summary
Problems solved by technology
Method used
Image
Examples
Embodiment Construction
[0029] The following and accompanying appendices illustrating the principles of the invention Figure 1 A detailed description of one or more embodiments of the invention is provided together. The invention is described in connection with such examples, but the invention is not limited to any example. The scope of the invention is limited only by the claims and the invention encompasses numerous alternatives, modifications and equivalents. In the following description, numerous specific details are set forth in order to provide a thorough understanding of the present invention. These details are provided for the purpose of example and the invention may be practiced according to the claims without some or all of these specific details.
[0030] As mentioned above, the deep learning-based planning sub-goal merging method provided by the present invention can reasonably determine the upper limit of the aggregate granularity, and the computer itself can identify the characterist...
PUM
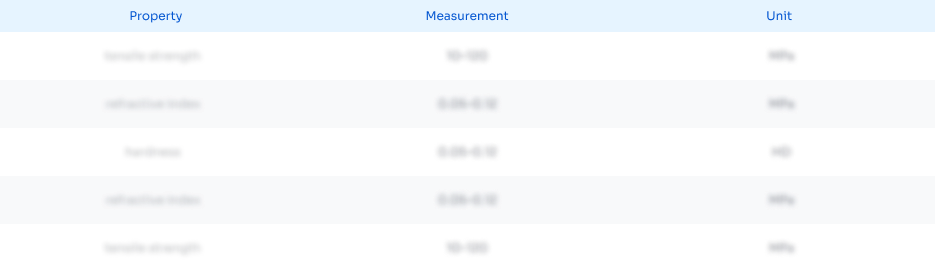
Abstract
Description
Claims
Application Information
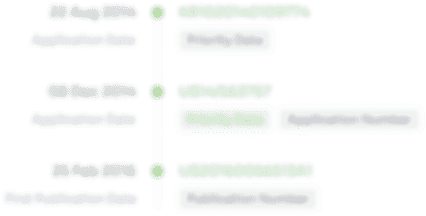
- R&D Engineer
- R&D Manager
- IP Professional
- Industry Leading Data Capabilities
- Powerful AI technology
- Patent DNA Extraction
Browse by: Latest US Patents, China's latest patents, Technical Efficacy Thesaurus, Application Domain, Technology Topic, Popular Technical Reports.
© 2024 PatSnap. All rights reserved.Legal|Privacy policy|Modern Slavery Act Transparency Statement|Sitemap|About US| Contact US: help@patsnap.com