Lightweight time convolution network for quick prediction of time series data
A time-series data and convolutional network technology, which is applied to biological neural network models, neural architectures, neural learning methods, etc., can solve the problems of reducing the amount of calculation and the large amount of calculation of the temporal convolutional network.
- Summary
- Abstract
- Description
- Claims
- Application Information
AI Technical Summary
Problems solved by technology
Method used
Image
Examples
Embodiment Construction
[0027] In order to make the object, technical solution and advantages of the present invention clearer, the present invention will be further described in detail below in conjunction with the accompanying drawings and embodiments. It should be understood that the specific embodiments described here are only used to explain the present invention, not to limit the present invention.
[0028] Such as Figure 2-Figure 5 As shown, in this embodiment, a lightweight temporal convolutional network oriented to rapid prediction of time series data includes a sequence x0,...,xT as input, aiming at predicting the predicted output y^T corresponding to xT, lightweight The temporal convolutional network formula is as follows:
[0029]
[0030] The lightweight temporal convolutional network mainly includes two major structures: causal convolution and step-size convolution. The overall framework of the idea is as follows: figure 2 shown. In causal convolution, the calculation value of e...
PUM
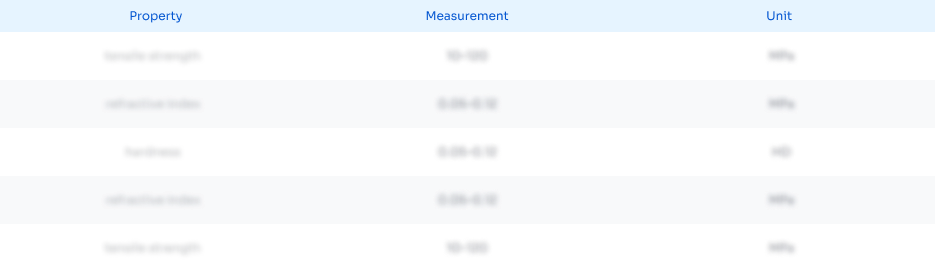
Abstract
Description
Claims
Application Information
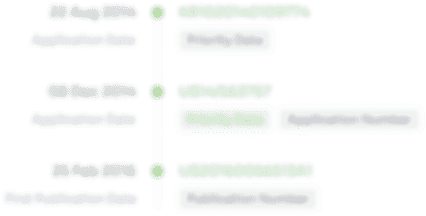
- R&D Engineer
- R&D Manager
- IP Professional
- Industry Leading Data Capabilities
- Powerful AI technology
- Patent DNA Extraction
Browse by: Latest US Patents, China's latest patents, Technical Efficacy Thesaurus, Application Domain, Technology Topic, Popular Technical Reports.
© 2024 PatSnap. All rights reserved.Legal|Privacy policy|Modern Slavery Act Transparency Statement|Sitemap|About US| Contact US: help@patsnap.com