Mechanical equipment fault diagnosis method based on deep learning
A technology of fault diagnosis and deep learning, applied in neural learning methods, computer components, program control, etc., can solve the problems of extracted features, insufficient accuracy, and failure to consider operating parameter data, inspection data, etc.
- Summary
- Abstract
- Description
- Claims
- Application Information
AI Technical Summary
Problems solved by technology
Method used
Image
Examples
Embodiment Construction
[0041] The present invention will be described below in conjunction with the accompanying drawings and specific embodiments. Unless otherwise specified, all are conventional methods.
[0042] Such as figure 1 and figure 2 As shown, a method for fault diagnosis of mechanical equipment based on deep learning includes the following steps:
[0043] Step S1: Collect and preprocess data from the main data source and secondary data source of mechanical equipment to obtain a data set, wherein the main data source is sensor monitoring data, and the secondary data source is operating status data and historical inspection data;
[0044] The sensor monitoring data is the measuring point information collected by the sensor in real time, which belongs to the time series data, so there is correlation in the time series. The selection of the fault diagnosis method of the present invention fully takes into account the time series correlation. First, obtain the long-term vibration signal of t...
PUM
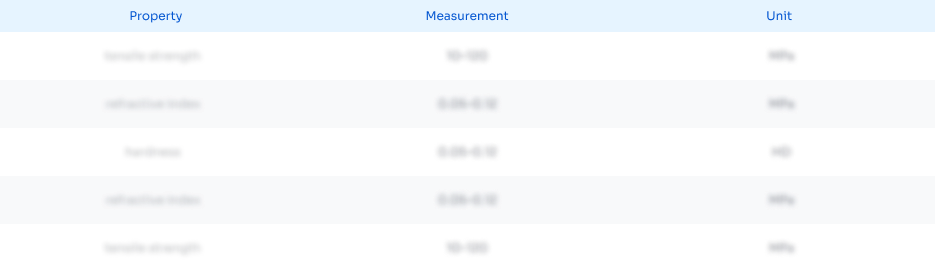
Abstract
Description
Claims
Application Information
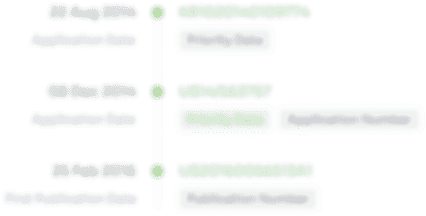
- R&D Engineer
- R&D Manager
- IP Professional
- Industry Leading Data Capabilities
- Powerful AI technology
- Patent DNA Extraction
Browse by: Latest US Patents, China's latest patents, Technical Efficacy Thesaurus, Application Domain, Technology Topic, Popular Technical Reports.
© 2024 PatSnap. All rights reserved.Legal|Privacy policy|Modern Slavery Act Transparency Statement|Sitemap|About US| Contact US: help@patsnap.com