Power distribution transformer area electricity sales accurate prediction method based on modal GRU learning network
A technology of learning network and forecasting method, which is applied in the field of accurate forecasting of power sales in distribution stations based on modal GRU learning network, can solve the problems of detailed decomposition of power data, poor forecasting effect, and failure to consider the impact, etc., to achieve The effect of improving accuracy, high accuracy, and good applicability
- Summary
- Abstract
- Description
- Claims
- Application Information
AI Technical Summary
Problems solved by technology
Method used
Image
Examples
Embodiment Construction
[0060] The present invention is described in further detail now in conjunction with accompanying drawing.
[0061] Such as figure 1 The shown method of accurate forecasting of electricity sales in distribution stations based on the modal GRU learning network includes the following steps:
[0062] Step 1: Obtain the historical data of electricity sales in the station area, and divide the test set and training set.
[0063] Step 2: Data preprocessing, complete the sampling time point to ensure its continuity, and use the average interpolation method to fill in the missing data of the sampling point.
[0064] Step 3: Determine the optimal mode number K of the variational mode decomposition (VMD) according to the center frequency of each modal component using an experimental method; this step specifically includes:
[0065] Step 3.1: Let the number of modes K=2, initialize the VMD parameters;
[0066] Step 3.2: Perform VMD decomposition on the time series of electricity sales t...
PUM
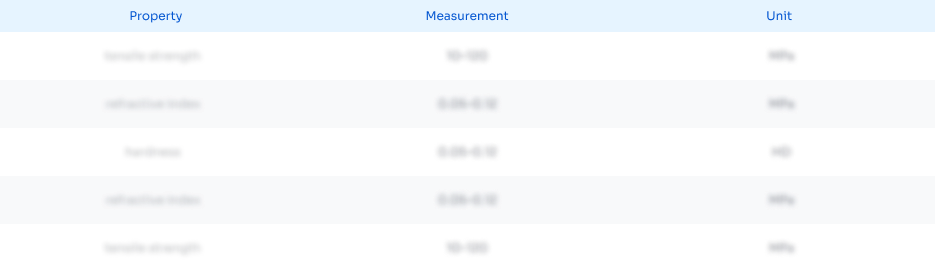
Abstract
Description
Claims
Application Information
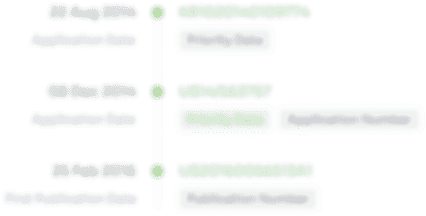
- R&D Engineer
- R&D Manager
- IP Professional
- Industry Leading Data Capabilities
- Powerful AI technology
- Patent DNA Extraction
Browse by: Latest US Patents, China's latest patents, Technical Efficacy Thesaurus, Application Domain, Technology Topic, Popular Technical Reports.
© 2024 PatSnap. All rights reserved.Legal|Privacy policy|Modern Slavery Act Transparency Statement|Sitemap|About US| Contact US: help@patsnap.com