Traffic flow prediction method based on feature reconstruction error
A traffic flow and reconstruction error technology, applied in the field of machine learning, can solve the problems of low feasibility of industrial application of traffic flow prediction, large calculation and space complexity, etc., and achieve the effect of stable model prediction and small space complexity
- Summary
- Abstract
- Description
- Claims
- Application Information
AI Technical Summary
Problems solved by technology
Method used
Image
Examples
Embodiment Construction
[0030] The present invention will be further described in detail below with reference to the accompanying drawings and embodiments. It should be noted that the following embodiments are intended to facilitate the understanding of the present invention, but do not limit it in any way.
[0031] Such as figure 1 As shown, a traffic flow prediction method based on feature reconstruction error, including:
[0032] S01. Initialize target machine learning network parameters.
[0033] The target machine learning network can be most commonly used deep neural network models such as space-time graph network (ST-GCN), or it can be a linear machine learning network such as least squares network (OLE).
[0034] In this embodiment, the least squares network (OLE) is used as the basic network to predict traffic flow as an example. At the same time, the initialization of the model parameters is obtained by Gaussian distribution sampling.
[0035] S02, initialize the feature correction weigh...
PUM
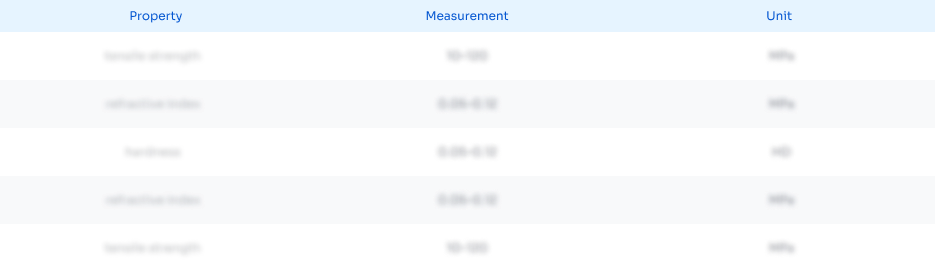
Abstract
Description
Claims
Application Information
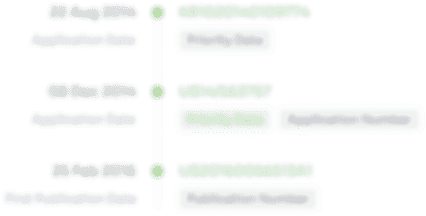
- R&D Engineer
- R&D Manager
- IP Professional
- Industry Leading Data Capabilities
- Powerful AI technology
- Patent DNA Extraction
Browse by: Latest US Patents, China's latest patents, Technical Efficacy Thesaurus, Application Domain, Technology Topic, Popular Technical Reports.
© 2024 PatSnap. All rights reserved.Legal|Privacy policy|Modern Slavery Act Transparency Statement|Sitemap|About US| Contact US: help@patsnap.com