Remote sensing image scene classification method based on semi-Bayesian deep learning based on Markov chain Monte Carlo and variational inference
A semi-Bayesian and deep learning technology, applied in the field of image processing, can solve problems such as redundant information, complex feature extraction, and poor classification performance, so as to enhance accuracy and robustness, avoid overfitting, and improve The effect on classification performance
- Summary
- Abstract
- Description
- Claims
- Application Information
AI Technical Summary
Problems solved by technology
Method used
Image
Examples
Embodiment Construction
[0083] The technical solutions of the present invention will be further described below with reference to the accompanying drawings and embodiments.
[0084] like figure 1 As shown, the technical scheme of the present invention is further described in detail as follows:
[0085] (1) Select the semi-Bayesian convolutional neural network as the remote sensing scene classification application, and build an eight-layer semi-Bayesian convolutional neural network model, in which the second convolutional layer, the fourth convolutional layer and the last three The weight parameters of the fully connected layer are represented by Gaussian distribution, and the weight parameters of the remaining first convolutional layer, third convolutional layer and fifth convolutional layer are represented by single point distribution .
[0086] (1.1) Build X={x i |i=1,2,...,N} is the input remote sensing image data sample, Y={y i |i=1,2,...,N} is the set of category labels corresponding to the ...
PUM
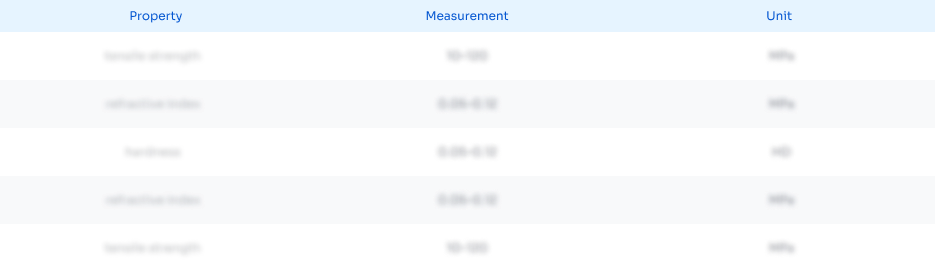
Abstract
Description
Claims
Application Information
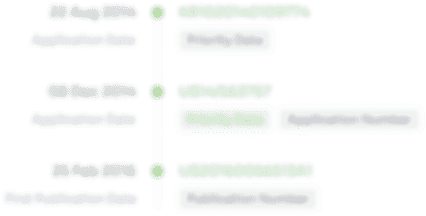
- R&D Engineer
- R&D Manager
- IP Professional
- Industry Leading Data Capabilities
- Powerful AI technology
- Patent DNA Extraction
Browse by: Latest US Patents, China's latest patents, Technical Efficacy Thesaurus, Application Domain, Technology Topic, Popular Technical Reports.
© 2024 PatSnap. All rights reserved.Legal|Privacy policy|Modern Slavery Act Transparency Statement|Sitemap|About US| Contact US: help@patsnap.com