Metabonomics marker kit for self-assembly detection of post-operation delirium and application
A technology of markers and kits, applied in the field of molecular biology, can solve problems such as time-consuming, high cost, and limited use, and achieve the effect of improving diagnostic sensitivity
- Summary
- Abstract
- Description
- Claims
- Application Information
AI Technical Summary
Problems solved by technology
Method used
Image
Examples
Embodiment 1
[0041] Peripheral blood was collected from the two groups of patients, and then the serum was separated and stored in a -80°C refrigerator. After the serum was thawed slowly at 4°C, 100 μL was taken respectively, and 400 μL of pre-cooled methanol / acetonitrile solution (1:1, v / v) was added, mixed by vortexing, allowed to stand at -20°C for 30 min, and then centrifuged at 14000 g for 20 min at 4°C. Take the supernatant after centrifugation, dry it in vacuum, add 100 μL acetonitrile aqueous solution (acetonitrile: water = 1:1, v / v) for mass spectrometry analysis, redissolve, vortex, centrifuge at 14000 g 4 °C for 15 min, and take the supernatant for analysis.
Embodiment 2
[0043] The following experiment was performed for detecting the expression level of the target metabolic marker from the serum of the patient.
[0044]Specifically, the patient samples in were separated using an Agilent 1290 Infinity LC ultra-high performance liquid chromatography system. Mobile phase: liquid A is 25mM ammonium formate + 0.08% FA aqueous solution, liquid B is 0.1% FA acetonitrile. The sample was placed in an autosampler at 4°C, the column temperature was 40°C, the flow rate was 250 μL / min, and the injection volume was 2 μL. The relevant liquid phase gradient is as follows: 0-12min, B liquid changes linearly from 90% to 70%; 12-18min, B liquid changes linearly from 70% to 50%; 18-25min, B liquid changes linearly from 50% to 40% ; 30-30.1min, B solution linearly changed from 40% to 90%; 30.1-37min, B solution maintained at 90%. A QC sample is set at every interval of a certain number of experimental samples in the sample queue to detect and evaluate the stab...
Embodiment 3
[0048] Using the identification of metabolites obtained from the , a model was constructed. The learning model was built by support vector mechanism (SVM) to classify the two groups. SVM models were constructed using the digit 2, 3, 5, 10, 20, 26 features respectively, which were constructed using radial basis kernel functions based on C classification. For the training and testing groups, each feature model is repeated 40 different samples. This in turn lists the selection frequencies of metabolites across all models. Finally, we selected ten characteristic substances to construct the final SVM model. Under this parameter, the prediction accuracy reaches 83.8%, and the area under the curve is 0.91, indicating that the model performs well. From this, ten metabolic markers (oleamide, monoglyceride 180 / 00 / 00, L-histidine, L-leucine, guanosine monophosphate, dihydrogen sphingosine-1 phosphite, hemolytic Phosphatidylcholine 150, L-glutamine, L-acylcarnitine, uric acid) can be ...
PUM
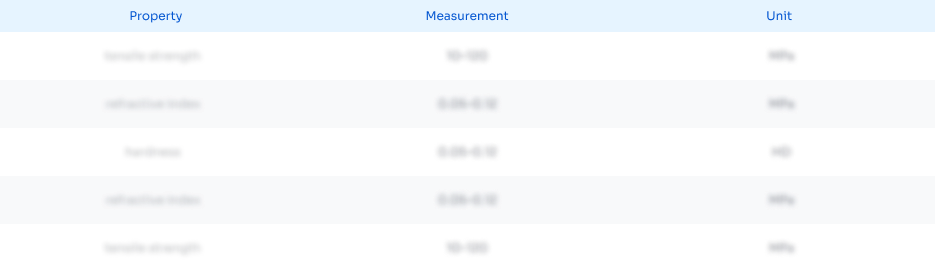
Abstract
Description
Claims
Application Information
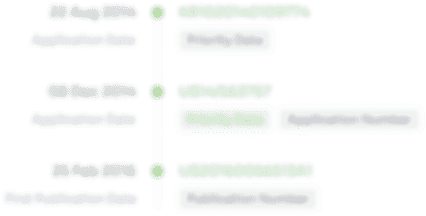
- Generate Ideas
- Intellectual Property
- Life Sciences
- Materials
- Tech Scout
- Unparalleled Data Quality
- Higher Quality Content
- 60% Fewer Hallucinations
Browse by: Latest US Patents, China's latest patents, Technical Efficacy Thesaurus, Application Domain, Technology Topic, Popular Technical Reports.
© 2025 PatSnap. All rights reserved.Legal|Privacy policy|Modern Slavery Act Transparency Statement|Sitemap|About US| Contact US: help@patsnap.com