Electronic equipment fault prediction method based on LSTM enhancement model
A technology for electronic equipment and fault prediction, which is applied in the field of neural network improvement, can solve problems such as decreased accuracy, and achieve the effect of improving memory ability
- Summary
- Abstract
- Description
- Claims
- Application Information
AI Technical Summary
Problems solved by technology
Method used
Image
Examples
Embodiment Construction
[0032] 1. Implementation principle of the existing LSTM model:
[0033] RNN is a neural network with a hidden layer with a self-connection relationship. Each node calculates the output at the current moment through the state at the current moment, and the state at the current moment is determined by the state at the previous moment and the input at the current moment, so as to realize Memory of time series data. LSTM continues the chain conduction structure of RNN, and adds four kinds of interaction gates on the basis of RNN. Such as figure 1 As shown, input (input gate), forget (forget gate), output (output gate) and cell (cell state) to solve the gradient explosion problem. Each LSTM node contains three inputs, namely the node state C at the last moment t-1 , the node output h at the last moment t-1 and the input x at the current moment t . The unique gate structure of LSTM contains a non-linear activation function sigmoid, which determines the amount of information pa...
PUM
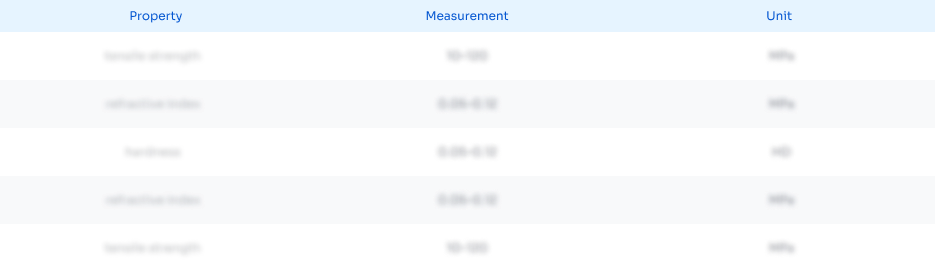
Abstract
Description
Claims
Application Information
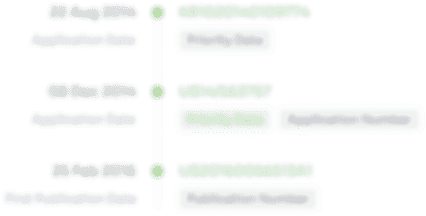
- R&D Engineer
- R&D Manager
- IP Professional
- Industry Leading Data Capabilities
- Powerful AI technology
- Patent DNA Extraction
Browse by: Latest US Patents, China's latest patents, Technical Efficacy Thesaurus, Application Domain, Technology Topic, Popular Technical Reports.
© 2024 PatSnap. All rights reserved.Legal|Privacy policy|Modern Slavery Act Transparency Statement|Sitemap|About US| Contact US: help@patsnap.com