Hyperspectral image classification method based on multi-scale spectral space convolutional neural network
A hyperspectral image and neural network technology, applied in the field of hyperspectral image classification, to achieve the effect of overcoming poor classification effect, improving classification ability, and improving classification accuracy
- Summary
- Abstract
- Description
- Claims
- Application Information
AI Technical Summary
Problems solved by technology
Method used
Image
Examples
Embodiment Construction
[0029] The embodiments and effects of the present invention will be further described below in conjunction with the accompanying drawings.
[0030] Refer to attached figure 1 , the implementation steps of this example include the following.
[0031] Step 1, input hyperspectral image.
[0032] A hyperspectral image is a three-dimensional data S ∈ R a×b×c , each band in the hyperspectral image corresponds to a two-dimensional matrix S in the three-dimensional data i ∈ R a×b , where, ∈ represents the belonging symbol, R represents the real number domain symbol, a represents the length of the hyperspectral image, b represents the width of the hyperspectral image, c represents the number of spectral bands in the hyperspectral image, and i represents the serial number of the spectral band in the hyperspectral image , i=1,2,...,c.
[0033] Step 2, obtain a collection of hyperspectral image blocks.
[0034] Perform 0 edge filling operation on the original 3D hyperspectral image ...
PUM
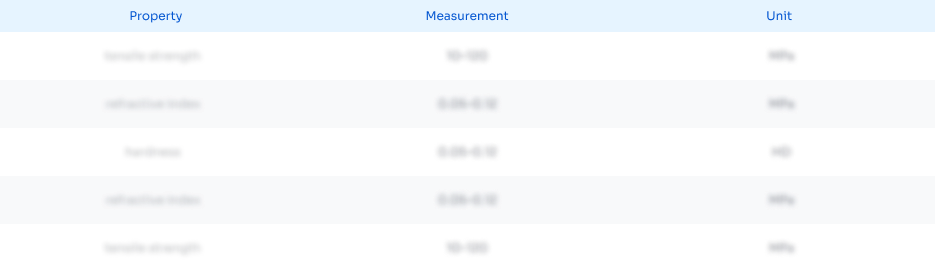
Abstract
Description
Claims
Application Information
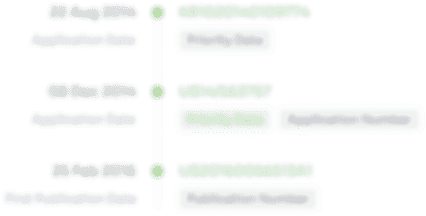
- Generate Ideas
- Intellectual Property
- Life Sciences
- Materials
- Tech Scout
- Unparalleled Data Quality
- Higher Quality Content
- 60% Fewer Hallucinations
Browse by: Latest US Patents, China's latest patents, Technical Efficacy Thesaurus, Application Domain, Technology Topic, Popular Technical Reports.
© 2025 PatSnap. All rights reserved.Legal|Privacy policy|Modern Slavery Act Transparency Statement|Sitemap|About US| Contact US: help@patsnap.com