Fine-grained image weak supervision target positioning method based on deep learning
A target positioning and deep learning technology, applied in the field of image-text target positioning in deep learning, can solve the problem of ignoring the fine-grained relationship between images and language descriptions, and achieve the effect of solving weakly supervised target positioning
- Summary
- Abstract
- Description
- Claims
- Application Information
AI Technical Summary
Problems solved by technology
Method used
Image
Examples
Embodiment Construction
[0022] The technical solution of the present invention will be further described below in conjunction with the accompanying drawings. figure 1 It is the overall flowchart of the method involved in the present invention.
[0023] Step 1, divide the dataset
[0024] The database in the implementation process of the method of the present invention comes from the public standard data set CUB-200-2011, which contains 11,788 color pictures of birds. The data set has 200 categories, each with about 60 images. The data set is a multi-label data set, and each picture has a corresponding ten-sentence language description. The image data set is divided into two parts, one part is used as a test sample set for testing the effect, and the other part is used as a training sample set for training the network model.
[0025] Step 2: Build an image and language two-way network model
[0026] The structure of the image-language localization network model is a two-way structure, one way is us...
PUM
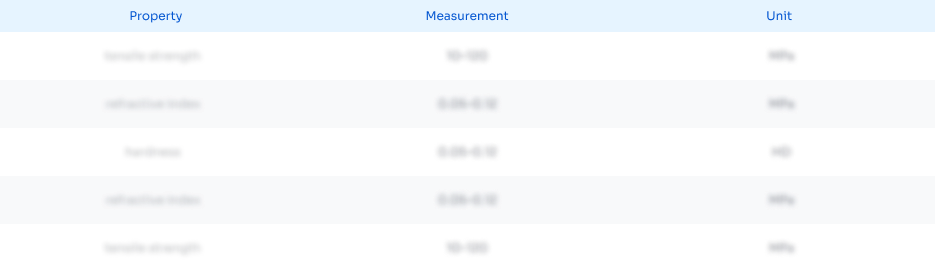
Abstract
Description
Claims
Application Information
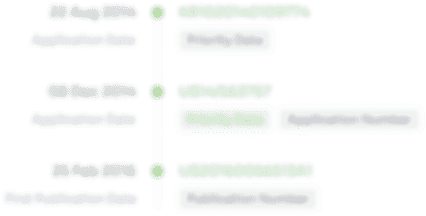
- R&D
- Intellectual Property
- Life Sciences
- Materials
- Tech Scout
- Unparalleled Data Quality
- Higher Quality Content
- 60% Fewer Hallucinations
Browse by: Latest US Patents, China's latest patents, Technical Efficacy Thesaurus, Application Domain, Technology Topic, Popular Technical Reports.
© 2025 PatSnap. All rights reserved.Legal|Privacy policy|Modern Slavery Act Transparency Statement|Sitemap|About US| Contact US: help@patsnap.com