Adenocarcinoma pathological image analysis method based on priori perception and multi-task learning
A multi-task learning, pathological image technology, applied in the field of adenocarcinoma pathological image analysis based on prior perception and multi-task learning, can solve problems such as lack of pathological level interpretation, and achieve high automatic diagnosis accuracy and robustness. The effect of strengthening and reducing physical strength
- Summary
- Abstract
- Description
- Claims
- Application Information
AI Technical Summary
Problems solved by technology
Method used
Image
Examples
specific Embodiment 1
[0044] combine figure 1 , the present invention proposes an adenocarcinoma pathological image analysis method based on prior perception and multi-task learning, the image analysis method comprising:
[0045]For the newly collected adenocarcinoma pathological image, the image block is extracted and the extracted image block is preprocessed and sent to the trained adenocarcinoma pathological image analysis model based on the multi-task learning method, the adenocarcinoma pathological image analysis model The model contains two parallel branches: glandular structure segmentation branch and benign and malignant automatic grading branch, and the prediction results of glandular structure and benign and malignant grading results are obtained at the same time.
[0046] Wherein, the segmentation result of the gland structure segmentation branch is transmitted to the benign and malignant automatic grading branch as prior information, and the benign and malignant grading branch is constr...
specific Embodiment 2
[0061] The present invention also mentions an adenocarcinoma pathological image analysis device based on prior perception and multi-task learning, the image analysis device includes an adenocarcinoma pathological image preprocessing module, an adenocarcinoma pathological image analysis model, and an adenocarcinoma pathological image analysis model building blocks.
[0062] The adenocarcinoma pathological image preprocessing module is used for performing corresponding preprocessing on newly acquired adenocarcinoma pathological images, and the preprocessing includes extracting image blocks and image block enhancement processing. Preferably, the adenocarcinoma pathological image preprocessing module can preprocess the sample image and the newly acquired image at the same time, reducing redundant functional modules.
[0063] The adenocarcinoma pathological image analysis model includes two parallel branches: a glandular structure segmentation branch and a benign and malignant auto...
specific Embodiment 3
[0069] The present invention also mentions an adenocarcinoma pathological image analysis system based on prior perception and multi-task learning, and the image analysis system includes a processor and a memory connected to each other.
[0070] A computer execution program is stored in the memory, and the processor executes the computer execution program stored in the memory to execute the adenocarcinoma pathological image analysis method based on prior perception and multi-task learning as described above.
[0071] Aspects of the invention are described in this disclosure with reference to the accompanying drawings, which show a number of illustrated embodiments. Embodiments of the present disclosure are not necessarily defined to include all aspects of the present invention. It should be appreciated that the various concepts and embodiments described above, as well as those described in more detail below, can be implemented in any of numerous ways, since the concepts and emb...
PUM
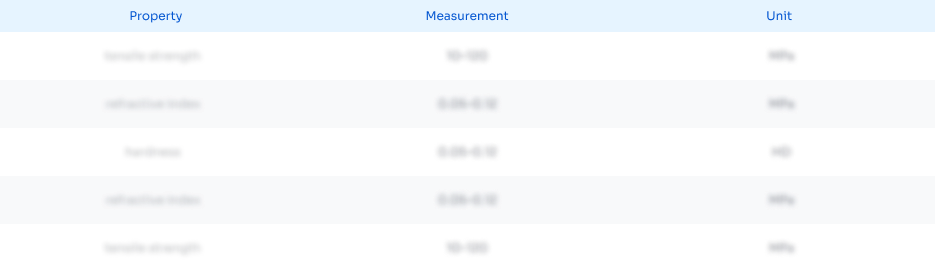
Abstract
Description
Claims
Application Information
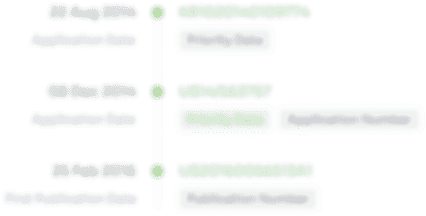
- R&D
- Intellectual Property
- Life Sciences
- Materials
- Tech Scout
- Unparalleled Data Quality
- Higher Quality Content
- 60% Fewer Hallucinations
Browse by: Latest US Patents, China's latest patents, Technical Efficacy Thesaurus, Application Domain, Technology Topic, Popular Technical Reports.
© 2025 PatSnap. All rights reserved.Legal|Privacy policy|Modern Slavery Act Transparency Statement|Sitemap|About US| Contact US: help@patsnap.com