Hybrid input method for solving electromagnetic inverse scattering problem based on deep learning
A hybrid input, deep learning technology, applied in neural learning methods, complex mathematical operations, biological neural network models, etc., can solve problems such as low computational cost, inability to distinguish, and inability to recover the dielectric constant of unknown scatterers
- Summary
- Abstract
- Description
- Claims
- Application Information
AI Technical Summary
Problems solved by technology
Method used
Image
Examples
Embodiment Construction
[0040] The present invention will be specifically introduced below in conjunction with the accompanying drawings and specific embodiments.
[0041] A mixed input method for solving electromagnetic inverse scattering problems based on deep learning, comprising the following steps:
[0042] Step 1, using the quantitative inversion method of fast imaging (ie non-iterative inversion method) to obtain approximate information of unknown scatterers, including contrast, permittivity, conductivity, etc.;
[0043] Step 2, use the qualitative inversion method of real-time imaging (direct sampling method DSM) to obtain qualitative information of unknown scatterers, including normalized value, shape, position and number, etc. (reconstruct the shape and position of unknown scatterers) ; The qualitative inversion method generally does not need to provide the material properties (permittivity, conductivity, etc.) The inside or outside of the unknown scatterer, according to the indicator func...
PUM
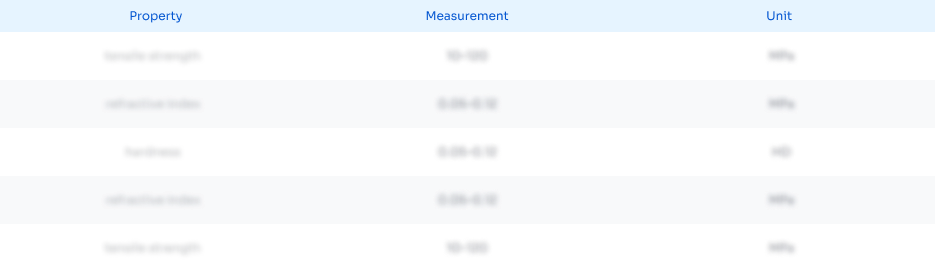
Abstract
Description
Claims
Application Information
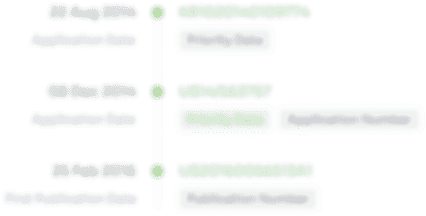
- R&D Engineer
- R&D Manager
- IP Professional
- Industry Leading Data Capabilities
- Powerful AI technology
- Patent DNA Extraction
Browse by: Latest US Patents, China's latest patents, Technical Efficacy Thesaurus, Application Domain, Technology Topic, Popular Technical Reports.
© 2024 PatSnap. All rights reserved.Legal|Privacy policy|Modern Slavery Act Transparency Statement|Sitemap|About US| Contact US: help@patsnap.com