Generative conference abstracting method based on graph convolutional neural network
A convolutional neural network and conference abstract technology, applied in the field of generative conference abstracts, which can solve the problem of ignoring the structural information of dialogue chapters
- Summary
- Abstract
- Description
- Claims
- Application Information
AI Technical Summary
Problems solved by technology
Method used
Image
Examples
specific Embodiment approach 1
[0028] Specific implementation mode one: combine figure 1 Describe this implementation mode. In this implementation mode, a generative conference summarization method based on graph convolutional neural network has a specific process as follows:
[0029] Step 1: Train the dialogue discourse structure parser, and mark the meeting data to obtain the dialogue discourse structure of the meeting;
[0030] Step 2: For each meeting in the meeting data set and the dialogue chapter structure of the meeting obtained in step 1, construct a meeting chapter structure diagram. The meeting chapter structure diagram includes all sentences in the meeting, all participants in the meeting, and Dialogue discourse structure between sentences;
[0031] Denote the conference chapter structure graph as G D =(V D ,E D , R D ), where v i ∈V D represent graph nodes, (v i ,r,v j )∈E D Represents the edge in the graph, r∈R D Represents the edge relationship in the graph;
[0032] Step 3: Use t...
specific Embodiment approach 2
[0038] Embodiment 2: This embodiment differs from Embodiment 1 in that: in the step 1, train the dialogue text structure parser, and mark the meeting data to obtain the dialogue text structure of the meeting; the specific process is:
[0039] Step 11. Use the existing STAC dataset [5] (Title: Discourse structure and dialogueacts in multiparty dialogue: The stac corpus, Authors: Nicholas Asher, Julie Hunter, Mathieu Morey, Farah Benamara, and Stergos Afantenos, Year: 2016) Training the existing dialogue structure parser Deep Sequential [6] (Title: Adeep sequential model for discourse parsing on multi-party dialogues, author: Zhouxing Shi and Minlie Huang, time: 2019, literature quoted from: Proceedings of the AAAI Conference on Artificial Intelligence), get the trained dialogue text structure parser Deep Sequential;
[0040] Step 1 and 2: Use the trained dialogue discourse structure parser Deep Sequential to mark the dialogue discourse structure of the AMI meeting data, and obt...
specific Embodiment approach 3
[0042] Specific embodiment three: what this embodiment is different from specific embodiment one or two is: utilize existing STAC data set to train existing dialogue discourse structure parser Deep Sequentia in the described step one by one, obtain the dialogue discourse structure analysis that has trained Deep Sequential; the specific process is:
[0043] The STAC dataset is a multiplayer chat dataset for English games. This corpus marks the semantic relationship between the basic semantic units (EDU) in the dialogue;
[0044] Said multiple persons are 3 or more persons.
[0045] According to statistics, STAC contains a total of 1091 dialogues, 10677 basic semantic units, and 11348 relational instances;
[0046] According to the task definition, at least 3 speakers participated in each dialogue. Each message sent by each speaker is usually 1-2 sentences each time, and each sentence is usually regarded as an elementary semantic unit (EDU) in multi-person dialogue discourse a...
PUM
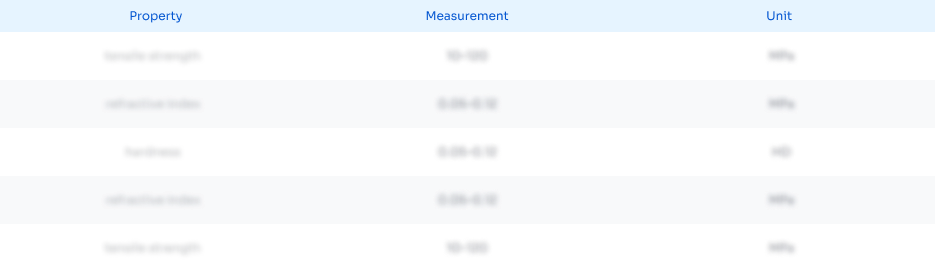
Abstract
Description
Claims
Application Information
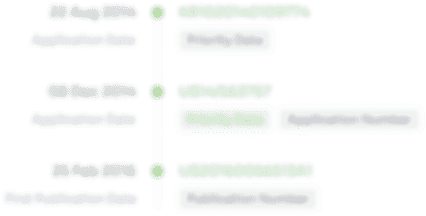
- R&D Engineer
- R&D Manager
- IP Professional
- Industry Leading Data Capabilities
- Powerful AI technology
- Patent DNA Extraction
Browse by: Latest US Patents, China's latest patents, Technical Efficacy Thesaurus, Application Domain, Technology Topic, Popular Technical Reports.
© 2024 PatSnap. All rights reserved.Legal|Privacy policy|Modern Slavery Act Transparency Statement|Sitemap|About US| Contact US: help@patsnap.com