Sliding bearing fault diagnosis method based on generative adversarial network and convolutional neural network
A convolutional neural network and sliding bearing technology, applied in the field of sliding bearing fault diagnosis based on generative confrontation network and convolutional neural network, can solve the increased workload and difficulty of fault diagnosis, the inability to achieve accurate diagnosis, and the number of samples required and other problems to achieve the effect of solving the lack of original data sets, speeding up training, and reducing dimensions
- Summary
- Abstract
- Description
- Claims
- Application Information
AI Technical Summary
Problems solved by technology
Method used
Image
Examples
Embodiment Construction
[0029] The following will clearly and completely describe the technical solutions in the embodiments of the present invention with reference to the accompanying drawings in the embodiments of the present invention. Obviously, the described embodiments are only some, not all, embodiments of the present invention. Based on the embodiments of the present invention, all other embodiments obtained by persons of ordinary skill in the art without creative efforts fall within the protection scope of the present invention.
[0030] Such as figure 1 As shown, a sliding bearing fault diagnosis method based on generative confrontation network and convolutional neural network, the method includes:
[0031] Step 1: At the sampling frequency f 1 The vibration signals of sliding bearings with no fault and different fault degrees under different fault conditions are collected, and the frequency f 2 Collect the bearing bush temperature corresponding to the vibration signal within this time pe...
PUM
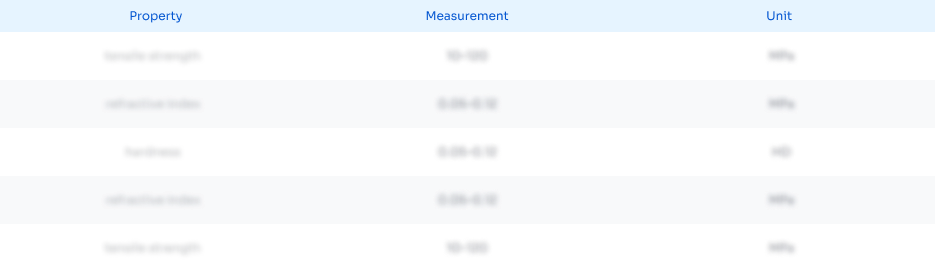
Abstract
Description
Claims
Application Information
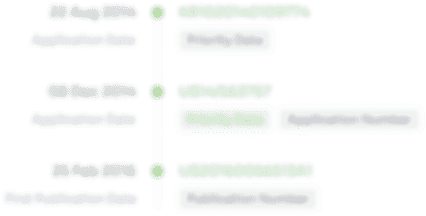
- R&D Engineer
- R&D Manager
- IP Professional
- Industry Leading Data Capabilities
- Powerful AI technology
- Patent DNA Extraction
Browse by: Latest US Patents, China's latest patents, Technical Efficacy Thesaurus, Application Domain, Technology Topic, Popular Technical Reports.
© 2024 PatSnap. All rights reserved.Legal|Privacy policy|Modern Slavery Act Transparency Statement|Sitemap|About US| Contact US: help@patsnap.com