Training method for improving robustness of multi-branch prediction single model in Internet of Things scene
A training method and multi-branch technology, applied in the computer field, can solve problems such as the inability to reflect the diversity of Internet of Things data, and achieve the effect of improving classification ability and robustness.
- Summary
- Abstract
- Description
- Claims
- Application Information
AI Technical Summary
Problems solved by technology
Method used
Image
Examples
Embodiment Construction
[0028] In order to make the object, technical solution and advantages of the present invention more clear, the present invention will be further described in detail below in conjunction with the accompanying drawings and embodiments.
[0029] The multi-branch prediction single model is structurally shaped like figure 1 .
[0030] In such a structured model, L ED The loss function makes the branch direct non-maximum prediction vectors classified as much as possible during training, so as to realize the overall diversity improvement effect of this model, thereby improving the robustness and the defense ability against adversarial samples. The LED loss function formula is:
[0031] L ED = L BE +γ·H(P * )+μ·L Ds
[0032] Where LBE is cross entropy, H(P * ) is the Shannon entropy, L DS is the separation measure of the non-maximum prediction vector, γ and μ are hyperparameters, representing the influence ability of the two parts.
[0033] In conventional training, the pre...
PUM
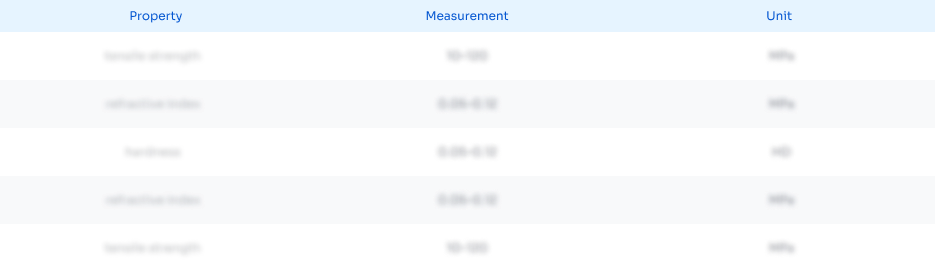
Abstract
Description
Claims
Application Information
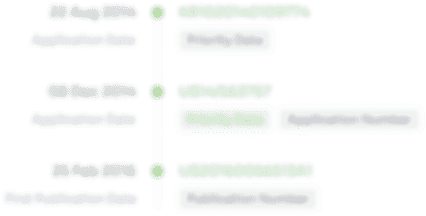
- R&D Engineer
- R&D Manager
- IP Professional
- Industry Leading Data Capabilities
- Powerful AI technology
- Patent DNA Extraction
Browse by: Latest US Patents, China's latest patents, Technical Efficacy Thesaurus, Application Domain, Technology Topic, Popular Technical Reports.
© 2024 PatSnap. All rights reserved.Legal|Privacy policy|Modern Slavery Act Transparency Statement|Sitemap|About US| Contact US: help@patsnap.com