Domain generalization and domain adaptive learning method based on data expansion consistency
An adaptive learning and consistency technology, applied in domain generalization and domain adaptive deep learning, can solve the problems of adaptive method model complexity, training difficulty, domain offset, etc.
- Summary
- Abstract
- Description
- Claims
- Application Information
AI Technical Summary
Problems solved by technology
Method used
Image
Examples
Embodiment
[0068] This example takes the image classification on the PACS dataset as an example. The PACS dataset is a domain generalization standard dataset constructed by the author of the literature (Deeper, Broader and Artier Domain Generalization, Da Li, ICCV2017). The dataset includes 4 different fields , which are photos (Photo), stick figures (Sketch), cartoon images (Cartoon) and art paintings (Art Painting), each domain contains images of 7 categories (respectively: "dog", "elephant", "giraffe", "guitar", "horse" and "person"), the total number of images in the dataset is 9991 frames. The embodiment is divided into domain generalization and domain adaptation. For domain generalization, each group of experiments uses three domains as the source domain D s , taking the remaining domain as the target domain D t , without using any information from the target domain during training. In domain adaptive learning, we also select three domains as the source domain D for each experime...
PUM
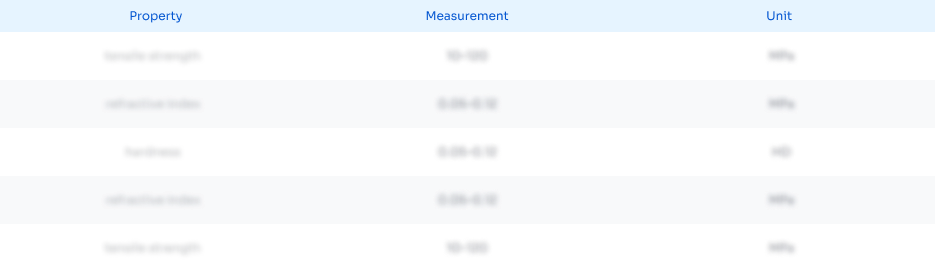
Abstract
Description
Claims
Application Information
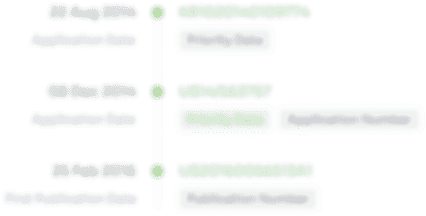
- R&D Engineer
- R&D Manager
- IP Professional
- Industry Leading Data Capabilities
- Powerful AI technology
- Patent DNA Extraction
Browse by: Latest US Patents, China's latest patents, Technical Efficacy Thesaurus, Application Domain, Technology Topic, Popular Technical Reports.
© 2024 PatSnap. All rights reserved.Legal|Privacy policy|Modern Slavery Act Transparency Statement|Sitemap|About US| Contact US: help@patsnap.com