Urban human flow mode detection method based on deep neural network graph encoder
A deep neural network and flow mode technology, applied in biological neural network models, neural architectures, instruments, etc., can solve problems such as lack of evaluation measures for topic models, difficult topic model capture, and time-sensitive urban data, so as to achieve effective urban area selection. , reduce the running time, the effect of good structural characteristics
- Summary
- Abstract
- Description
- Claims
- Application Information
AI Technical Summary
Problems solved by technology
Method used
Image
Examples
Embodiment
[0109] The city object studied in this embodiment is the Shanghai area with a range of 10000m×10000m, and a large amount of shared bicycle data is collected to test the method of the present invention. The collected data contains 957,357,367 riding records, and each record specifies a bicycle ID, pick-up location (starting point latitude and longitude), pick-up time (starting point time), parking location (end point longitude and latitude), and parking time (end point time). distributed as figure 2 As shown, the denser the points in the figure, the greater the density of bicycles, and the contrast figure 2 (a), figure 2 (b) It can be seen that the bicycle density differs significantly in different time periods.
[0110] based on figure 2 The flow data in (b), select respectively the suburbs in the city area and two districts of the city center as the center, and the circular range of 2000 meters radius around it as the research area, utilize the improved OPTICS algorith...
PUM
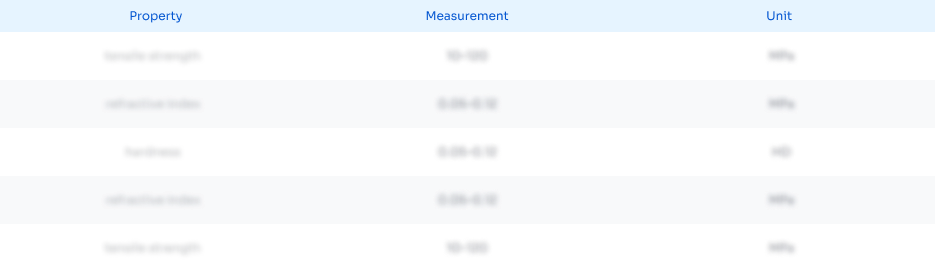
Abstract
Description
Claims
Application Information
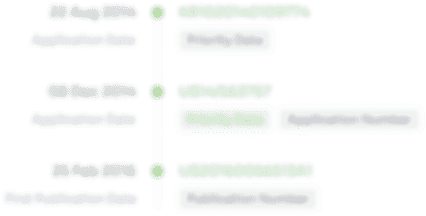
- R&D Engineer
- R&D Manager
- IP Professional
- Industry Leading Data Capabilities
- Powerful AI technology
- Patent DNA Extraction
Browse by: Latest US Patents, China's latest patents, Technical Efficacy Thesaurus, Application Domain, Technology Topic, Popular Technical Reports.
© 2024 PatSnap. All rights reserved.Legal|Privacy policy|Modern Slavery Act Transparency Statement|Sitemap|About US| Contact US: help@patsnap.com