Convolution kernel similarity pruning-based recurrent neural network model compression method
A technology of cyclic neural network and compression method, which is applied in the field of cyclic neural network model compression based on convolution kernel similarity pruning, which can solve the problems of reducing model size, accuracy loss, and less impact on accuracy, etc., to improve reasoning Effects of speed, maintaining regularity, and reducing loss of accuracy
- Summary
- Abstract
- Description
- Claims
- Application Information
AI Technical Summary
Problems solved by technology
Method used
Image
Examples
Embodiment Construction
[0045] The present invention will be further described below in conjunction with specific examples.
[0046] Such as figure 1 As shown, step 1, load the pre-trained cyclic neural network model into the compressed cyclic neural network for training, set the parameters of the pre-trained cyclic neural network model to be consistent with the parameters in the compressed cyclic neural network, and obtain the cyclic neural network initialized with the weight matrix Model.
[0047] Prepare the pre-trained cyclic neural network model to be compressed. Set the data set, configuration parameters, given norm pruning rate P1 and similarity pruning rate P2 to be consistent with the parameters in the compressed cyclic neural network, and set the pre-trained cyclic neural network model to be compressed Load into a compressed recurrent neural network for training.
[0048] Such as figure 2As shown, the data set used is WikiText-2 English thesaurus data, and each vocabulary also retains t...
PUM
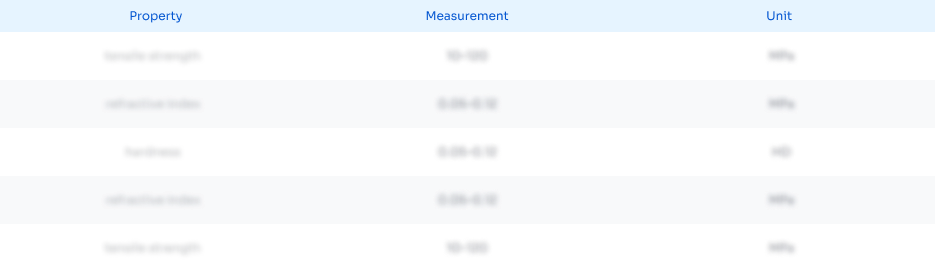
Abstract
Description
Claims
Application Information
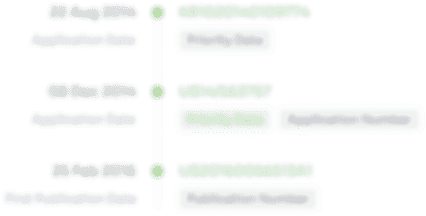
- Generate Ideas
- Intellectual Property
- Life Sciences
- Materials
- Tech Scout
- Unparalleled Data Quality
- Higher Quality Content
- 60% Fewer Hallucinations
Browse by: Latest US Patents, China's latest patents, Technical Efficacy Thesaurus, Application Domain, Technology Topic, Popular Technical Reports.
© 2025 PatSnap. All rights reserved.Legal|Privacy policy|Modern Slavery Act Transparency Statement|Sitemap|About US| Contact US: help@patsnap.com