Neural network optimization method for solving and lifting adjacent operator machine
A neural network and optimization method technology, applied in neural learning methods, biological neural network models, etc., can solve problems such as learning efficiency difficulties, achieve the effects of avoiding gradient disappearance or explosion, high accuracy, and improving training effects
- Summary
- Abstract
- Description
- Claims
- Application Information
AI Technical Summary
Problems solved by technology
Method used
Image
Examples
Embodiment Construction
[0062] Below in conjunction with accompanying drawing, further describe the present invention through embodiment, but do not limit the scope of the present invention in any way.
[0063] The present invention provides a neural network optimization method for solving the lifting adjacent operator machine. In the training of the forward neural network, a new method of block coordinate descent is used to solve the LPOM model, and the convergence is guaranteed for each sub-problem in the LPOM model. , which can update variables in parallel to improve the accuracy of neural network training without occupying additional memory space. The neural network optimization method proposed by the invention can be applied to specific tasks such as image recognition, speech recognition and natural language processing.
[0064] The following takes image recognition as an example to describe the specific implementation and compare it with the current best results. The method of the present inve...
PUM
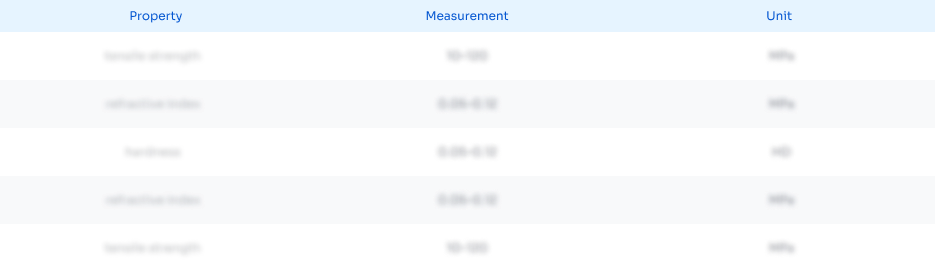
Abstract
Description
Claims
Application Information
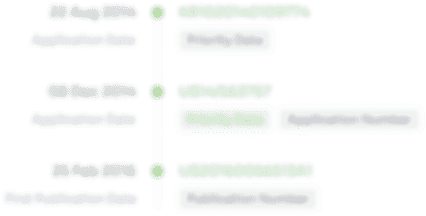
- R&D Engineer
- R&D Manager
- IP Professional
- Industry Leading Data Capabilities
- Powerful AI technology
- Patent DNA Extraction
Browse by: Latest US Patents, China's latest patents, Technical Efficacy Thesaurus, Application Domain, Technology Topic, Popular Technical Reports.
© 2024 PatSnap. All rights reserved.Legal|Privacy policy|Modern Slavery Act Transparency Statement|Sitemap|About US| Contact US: help@patsnap.com