Attention-based CNN category activation graph generation method
A technology of attention and categories, applied in neural learning methods, character and pattern recognition, biological neural network models, etc., can solve the problems of inability to explain classification results, inability to locate the largest contribution of image classification results, etc., to achieve flexible use and visual effects Good results
- Summary
- Abstract
- Description
- Claims
- Application Information
AI Technical Summary
Problems solved by technology
Method used
Image
Examples
Embodiment Construction
[0040] The present invention will be further explained below in conjunction with accompanying drawing and specific embodiment:
[0041] (1) Feature map visualization, CAM and Grad-CAM analysis
[0042] Convolutional neural networks are good at representation learning. Hidden layer filters can be regarded as different types of feature extractors, which perform hierarchical feature extraction and representation on input images. The feature maps encoded by the hidden layers of different levels focus on different aspects. The feature maps of the lower layers learn contour features such as edges and textures, while the feature maps of the higher layers learn local features such as target details. The higher the neuron in the convolutional layer, the richer the semantic information it contains, and the more distinguishable it is for objects, scenes and other targets. Therefore, the feature map of CNN can be regarded as the feature space of the input image, especially the high-level...
PUM
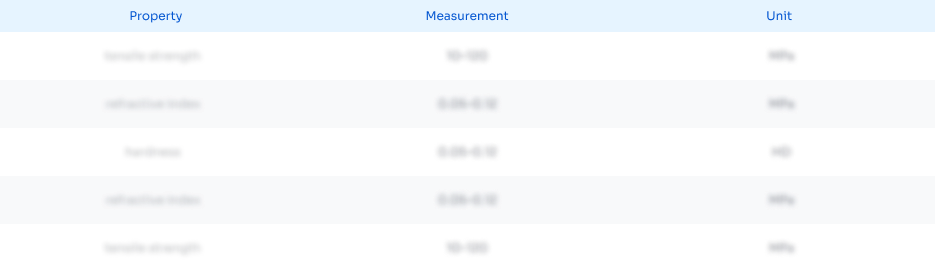
Abstract
Description
Claims
Application Information
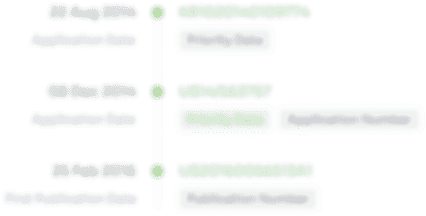
- R&D Engineer
- R&D Manager
- IP Professional
- Industry Leading Data Capabilities
- Powerful AI technology
- Patent DNA Extraction
Browse by: Latest US Patents, China's latest patents, Technical Efficacy Thesaurus, Application Domain, Technology Topic, Popular Technical Reports.
© 2024 PatSnap. All rights reserved.Legal|Privacy policy|Modern Slavery Act Transparency Statement|Sitemap|About US| Contact US: help@patsnap.com