Image segmentation method for enhancing edge and detail information by utilizing feature fusion
A technology of feature fusion and detail information, applied in the field of computer vision, can solve the problems of loss of position information and boundary detail information, the accuracy of segmentation needs to be improved, and the process is irreversible, so as to achieve clear boundaries and details, improve the segmentation effect, and improve the average accuracy. Effect
- Summary
- Abstract
- Description
- Claims
- Application Information
AI Technical Summary
Problems solved by technology
Method used
Image
Examples
Embodiment Construction
[0052] The specific implementation manners of the present invention will be further described in detail below in conjunction with the accompanying drawings and embodiments. The following examples are used to illustrate the present invention, but are not intended to limit the scope of the present invention.
[0053] In this embodiment, an image segmentation method using feature fusion to enhance edge and detail information, such as figure 1 shown, including the following steps:
[0054] Step 1: Process the images in the training data set to obtain images with uniform resolution;
[0055] Step 1.1: Scale and crop the images in the training dataset so that the input images have a uniform size;
[0056] Step 1.2: Fix the resolution of the input image to 360×480;
[0057] Step 2: Input the image into the encoding structure for feature extraction; the encoding structure is the same as the SegNet network, using the first 13 layers of VGG-16, and adding the maximum pooling index to...
PUM
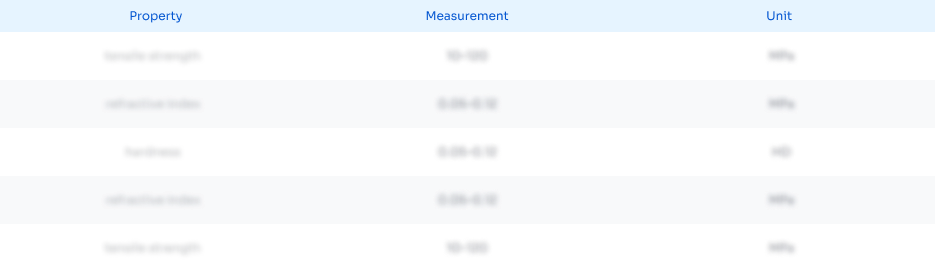
Abstract
Description
Claims
Application Information
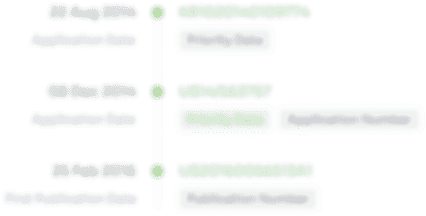
- R&D Engineer
- R&D Manager
- IP Professional
- Industry Leading Data Capabilities
- Powerful AI technology
- Patent DNA Extraction
Browse by: Latest US Patents, China's latest patents, Technical Efficacy Thesaurus, Application Domain, Technology Topic.
© 2024 PatSnap. All rights reserved.Legal|Privacy policy|Modern Slavery Act Transparency Statement|Sitemap