Thoracic cavity multi-organ segmentation method based on cascade residual full convolutional network
A fully convolutional network and multi-organ technology, applied in the field of thoracic multi-organ segmentation based on cascaded residual full convolutional network, can solve the problem of excessive calculation of 3D network, inability to learn spatial information, and easy to be limited by hardware capabilities and other problems, to achieve the effect of stable training process, good training effect, and accurate segmentation
- Summary
- Abstract
- Description
- Claims
- Application Information
AI Technical Summary
Problems solved by technology
Method used
Image
Examples
Embodiment Construction
[0035] The present invention will be further described in detail below with reference to the accompanying drawings and embodiments. It should be noted that the following embodiments are intended to facilitate the understanding of the present invention, but do not limit it in any way.
[0036] In the specific embodiment of the present invention, the multi-organ segmentation of thoracic CT is taken as an example to illustrate, but the present invention is not limited thereto. Non-essential improvements and adjustments made by those skilled in the art under the core guiding ideology of the present invention still belong to protection scope of the present invention.
[0037] Step 1: Data preprocessing in the rough segmentation stage
[0038]This article uses chest CT images. In order to remove excessive impurities and improve the contrast between organs and the background, in the rough segmentation stage, we cut off the range of CT values, set the window level to -500, and the win...
PUM
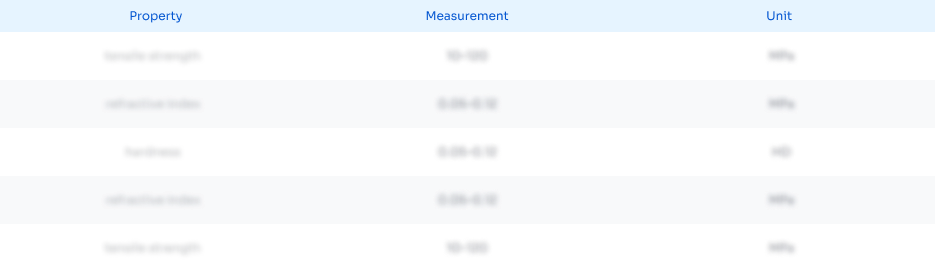
Abstract
Description
Claims
Application Information
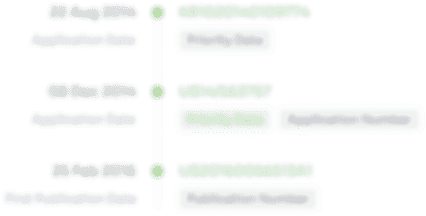
- R&D Engineer
- R&D Manager
- IP Professional
- Industry Leading Data Capabilities
- Powerful AI technology
- Patent DNA Extraction
Browse by: Latest US Patents, China's latest patents, Technical Efficacy Thesaurus, Application Domain, Technology Topic, Popular Technical Reports.
© 2024 PatSnap. All rights reserved.Legal|Privacy policy|Modern Slavery Act Transparency Statement|Sitemap|About US| Contact US: help@patsnap.com