Image splicing tampering positioning method based on full convolutional neural network
A convolutional neural network and image stitching technology, applied in the field of deep learning, can solve problems such as non-end-to-end structure, reduced model training speed, and difficulty in training deep and complex models of positioning methods.
- Summary
- Abstract
- Description
- Claims
- Application Information
AI Technical Summary
Problems solved by technology
Method used
Image
Examples
Embodiment Construction
[0069] The technical solutions of the present invention will be described in detail below in conjunction with the accompanying drawings.
[0070] The present invention uses deep learning technology to improve the prediction accuracy of splicing and tampering positioning. In deep learning technology, the one that is more suitable for processing visual information is the deep convolutional neural network, which is a method of supervised learning; residuals are also designed in this embodiment. The module reduces the difficulty of model training and speeds up the convergence speed; finally, the post-processing based on the conditional random field is used to optimize the prediction effect.
[0071] A method of image mosaic tampering localization based on fully convolutional neural network, such as figure 1 shown, including the following steps:
[0072] Step 1: Establish a stitched tampered image library, including training images and test images;
[0073] Step 2: Initialize the...
PUM
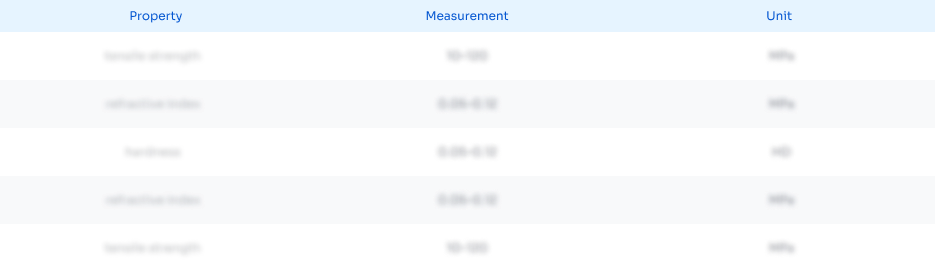
Abstract
Description
Claims
Application Information
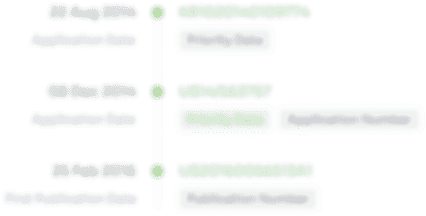
- Generate Ideas
- Intellectual Property
- Life Sciences
- Materials
- Tech Scout
- Unparalleled Data Quality
- Higher Quality Content
- 60% Fewer Hallucinations
Browse by: Latest US Patents, China's latest patents, Technical Efficacy Thesaurus, Application Domain, Technology Topic, Popular Technical Reports.
© 2025 PatSnap. All rights reserved.Legal|Privacy policy|Modern Slavery Act Transparency Statement|Sitemap|About US| Contact US: help@patsnap.com