Industrial steam quantity prediction method and device based on machine learning
A technology of machine learning and prediction methods, applied in neural learning methods, neural architectures, biological neural network models, etc., can solve problems such as difficult to achieve results, difficult to construct significant features of prediction models, and little improvement in the accuracy of prediction results, etc., to achieve The effect of high accuracy
- Summary
- Abstract
- Description
- Claims
- Application Information
AI Technical Summary
Problems solved by technology
Method used
Image
Examples
Embodiment 1
[0058] Attached figure 1 This embodiment proposes an industrial steam volume prediction method based on machine learning. The prediction method is based on the historical operating condition data collected by the boiler sensor and the actual exhaust steam volume. First, the historical operating condition data is decomposed by time series to obtain the historical operating condition The inherent trend and period information of the data are two characteristic data, and then the LSTM algorithm is used to learn the inherent trend and period information of the historical operating condition data, and then the LSTM algorithm prediction model is constructed with the aid of the actual emission training corresponding to the historical operating condition data. Finally, The newly collected working condition data of the boiler sensor is decomposed into the LSTM algorithm prediction model after time series decomposition, and the predicted steam volume of the boiler can be output.
[0059] In ...
Embodiment 2
[0080] Attached figure 2 In this embodiment, an industrial steam volume prediction device based on machine learning is proposed, and the prediction device includes:
[0081] The collection module 1 is used to collect the historical working condition data collected by the boiler sensor, the actual amount of steam discharged, and the newly collected working condition data of the boiler sensor;
[0082] Time series decomposition module 2, used to decompose the collected historical working condition data and new working condition data in time series, and obtain the two characteristic data of internal trend and period information of historical working condition data and new working condition data;
[0083] LSTM algorithm module 3, used to learn the inherent trend and cycle information of historical working condition data;
[0084] The training building module 4 is used to input the actual emissions corresponding to the historical working condition data into the LSTM algorithm module 3 to ...
PUM
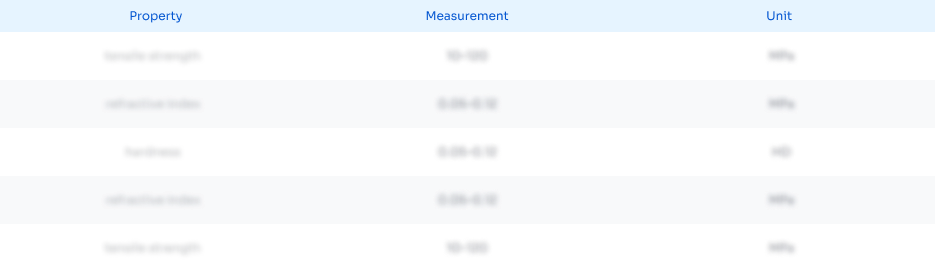
Abstract
Description
Claims
Application Information
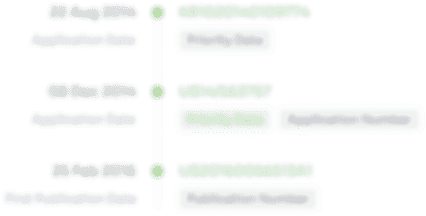
- Generate Ideas
- Intellectual Property
- Life Sciences
- Materials
- Tech Scout
- Unparalleled Data Quality
- Higher Quality Content
- 60% Fewer Hallucinations
Browse by: Latest US Patents, China's latest patents, Technical Efficacy Thesaurus, Application Domain, Technology Topic, Popular Technical Reports.
© 2025 PatSnap. All rights reserved.Legal|Privacy policy|Modern Slavery Act Transparency Statement|Sitemap|About US| Contact US: help@patsnap.com