Lithium ion battery remaining life prediction method based on fusion of gating circulation unit neural network and Kalman filtering model
A lithium-ion battery and Kalman filter technology, which is applied in biological neural network models, neural learning methods, and electrical measurement, can solve the problems of low adaptability to different working conditions and poor fitting ability of nonlinear degradation processes, etc. The effect of small noise
- Summary
- Abstract
- Description
- Claims
- Application Information
AI Technical Summary
Problems solved by technology
Method used
Image
Examples
specific Embodiment approach 1
[0027] Specific implementation mode one: refer to figure 1 Specifically explain this embodiment, the method for predicting the remaining life of a lithium-ion battery based on the fusion of the gated recurrent unit neural network and the Kalman filter model described in this embodiment, takes an 18650 battery with a rated capacity of 2200mAh for testing, and the specific steps of the test are as follows:
[0028] Step 1: Construct a data set using the battery capacity data in each charge and discharge cycle of the lithium-ion battery for training, and use the data in the data set as training data.
[0029] Step 2, use the training data to construct the training set of the GRU model, the training set is expressed as [xtrain, ytrain], where:
[0030]
[0031] In the above formula, Cap l Indicates the capacity of the first battery, Indicates the number of charge and discharge cycles corresponding to when the capacity of the first battery degrades to 80% of the rated capacit...
PUM
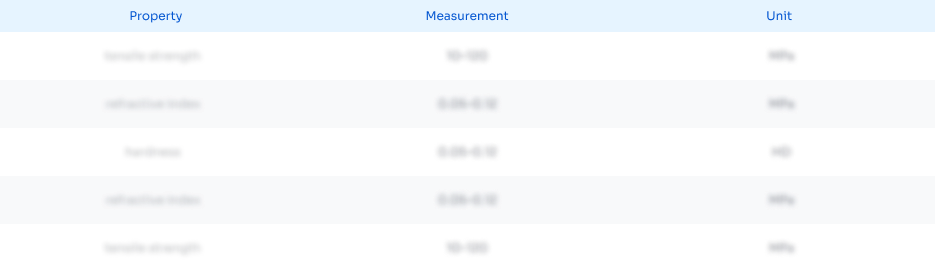
Abstract
Description
Claims
Application Information
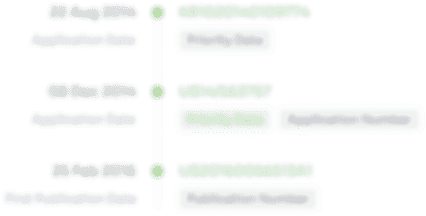
- R&D
- Intellectual Property
- Life Sciences
- Materials
- Tech Scout
- Unparalleled Data Quality
- Higher Quality Content
- 60% Fewer Hallucinations
Browse by: Latest US Patents, China's latest patents, Technical Efficacy Thesaurus, Application Domain, Technology Topic, Popular Technical Reports.
© 2025 PatSnap. All rights reserved.Legal|Privacy policy|Modern Slavery Act Transparency Statement|Sitemap|About US| Contact US: help@patsnap.com