Motor vehicle pollution discharge monitoring node deployment method based on active space-time diagram convolution
A technology for monitoring nodes and space-time diagrams, applied in prediction, complex mathematical operations, biological neural network models, etc., can solve the problems of limited quantity, high installation and maintenance costs of monitoring nodes, and achieve the effect of improving accuracy
- Summary
- Abstract
- Description
- Claims
- Application Information
AI Technical Summary
Problems solved by technology
Method used
Image
Examples
Embodiment Construction
[0048] The following is attached figure 1 To further illustrate the present invention, the method of the present invention includes the following steps:
[0049] Step 1: Feature extraction for structural sequence data
[0050] Use the idea of attention mechanism for reference to process the adjacency matrix to obtain the adaptive adjacency matrix:
[0051]
[0052] Among them, A is the adjacency matrix, I N Is the identity matrix, W embed Is a learnable embedding matrix, o is element-wise multiplication, and ReLU is a linear rectification function.
[0053] Send the adaptive adjacency matrix to the graph convolutional network to obtain the adaptive graph convolutional network, which is
[0054]
[0055] Where H (l) For l th Layer nonlinear transformation output, σ(·) is the activation function, H (0) =X is the feature input.
[0056] Adaptive graph convolution can adaptively adjust the weight of edges according to the graph structure and the characteristics of each node, can lear...
PUM
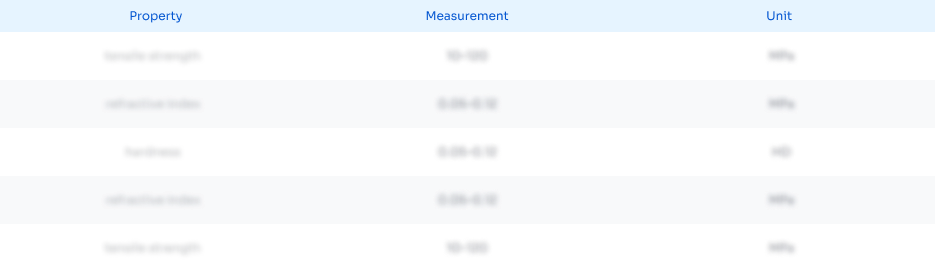
Abstract
Description
Claims
Application Information
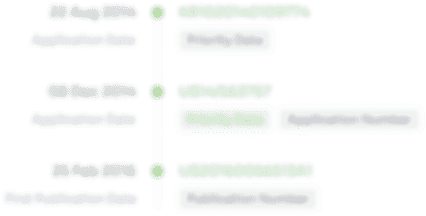
- Generate Ideas
- Intellectual Property
- Life Sciences
- Materials
- Tech Scout
- Unparalleled Data Quality
- Higher Quality Content
- 60% Fewer Hallucinations
Browse by: Latest US Patents, China's latest patents, Technical Efficacy Thesaurus, Application Domain, Technology Topic, Popular Technical Reports.
© 2025 PatSnap. All rights reserved.Legal|Privacy policy|Modern Slavery Act Transparency Statement|Sitemap|About US| Contact US: help@patsnap.com