CNN and transfer learning-based disease intelligent identification method and system
A technology of intelligent identification and transfer learning, applied in the field of intelligent identification, can solve the problems of increasing the complexity of the model and development cost, occupying a large amount of running memory and computing time, and poor computing performance, achieving fast computing speed, low image quality requirements, Good universal effect
- Summary
- Abstract
- Description
- Claims
- Application Information
AI Technical Summary
Problems solved by technology
Method used
Image
Examples
no. 1 example
[0054] In this embodiment, an intelligent disease identification system based on CNN and transfer learning is an algorithm model combining convolutional neural network and transfer learning to realize image intelligent diagnosis of common plant diseases. The plant in the present embodiment is example with corn, and this system is with corn common 10 classes (big spot, small spot, round spot, gray spot, stem rot, common rust, silk smut, ear rot disease, Curvularia leaf spot, sheath blight) diseases as the research object, according to the identification results, this system can accurately identify 10 common corn diseases, the correct rate can reach more than 90%, which provides an effective way for the prevention and control of corn diseases technical support.
[0055] See figure 1 , figure 1 It is a flowchart of the architecture of the identification system in this embodiment, which is mainly divided into three layers:
[0056] Service layer: It is used to display the resul...
PUM
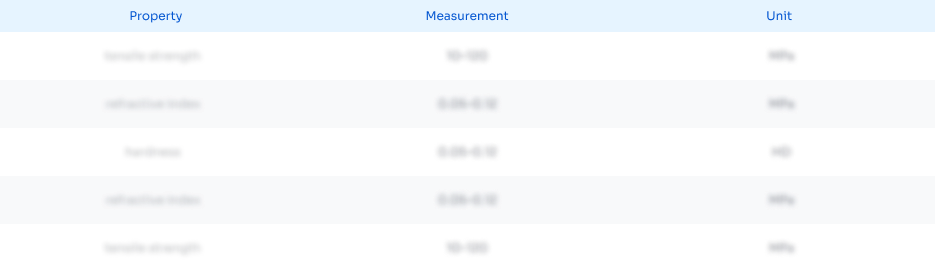
Abstract
Description
Claims
Application Information
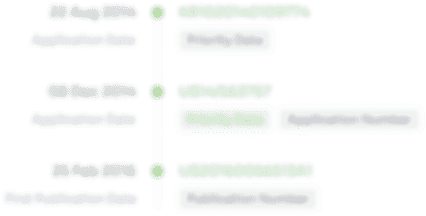
- R&D Engineer
- R&D Manager
- IP Professional
- Industry Leading Data Capabilities
- Powerful AI technology
- Patent DNA Extraction
Browse by: Latest US Patents, China's latest patents, Technical Efficacy Thesaurus, Application Domain, Technology Topic, Popular Technical Reports.
© 2024 PatSnap. All rights reserved.Legal|Privacy policy|Modern Slavery Act Transparency Statement|Sitemap|About US| Contact US: help@patsnap.com