A deep learning method for flow abnormity detection of an intelligent power grid server
A smart grid and traffic anomaly technology, applied in the direction of instruments, electrical components, biological neural network models, etc., can solve problems such as difficulty in distinguishing network anomalies, difficulties in anomaly detection and troubleshooting, and data communication security cannot be guaranteed, etc., to achieve enhanced The effect of adaptability
- Summary
- Abstract
- Description
- Claims
- Application Information
AI Technical Summary
Problems solved by technology
Method used
Image
Examples
Embodiment
[0025] This example uses figure 1 A deep neural network is shown to model the normal behavior of a target variable for a data server in a smart grid. For multi-concurrent servers based on the TCP protocol, TCP traffic accounts for the majority of the overall traffic, and many attacks are also directed at TCP, so this embodiment considers the characteristic variables reflecting TCP traffic, and the variable description is as shown in Table 1.
[0026] Table 1 Feature List
[0027]
[0028]
[0029] In this embodiment, the downlink traffic of the server is used as a target variable representing the normal behavior of network traffic, and other variables are used as related variables for reference in estimating the target variable. The historical detection data of a smart grid server is selected. The measured values of the data set are all 1-minute average values. A total of 4 weeks of data are selected. According to the log record information, it can be known that there...
PUM
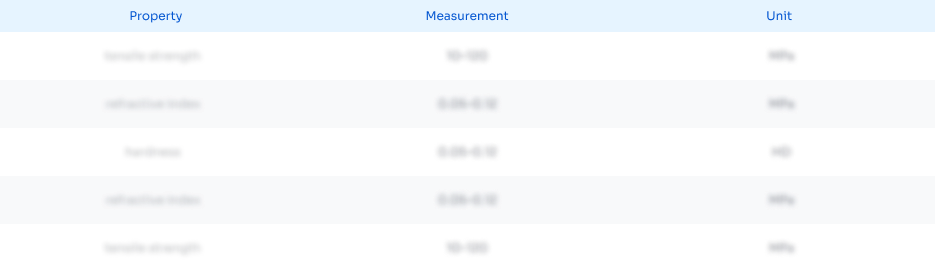
Abstract
Description
Claims
Application Information
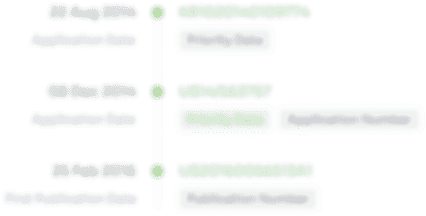
- R&D Engineer
- R&D Manager
- IP Professional
- Industry Leading Data Capabilities
- Powerful AI technology
- Patent DNA Extraction
Browse by: Latest US Patents, China's latest patents, Technical Efficacy Thesaurus, Application Domain, Technology Topic, Popular Technical Reports.
© 2024 PatSnap. All rights reserved.Legal|Privacy policy|Modern Slavery Act Transparency Statement|Sitemap|About US| Contact US: help@patsnap.com