Robust service quality prediction and guarantee method based on deep learning in SDN
A technology of deep learning and quality of service, applied in the field of control layer in software-defined network, can solve problems such as the impact of prediction accuracy, lack of credibility of attribute weights, and intricate network data
- Summary
- Abstract
- Description
- Claims
- Application Information
AI Technical Summary
Problems solved by technology
Method used
Image
Examples
Embodiment Construction
[0040] The technical solution of the present invention will be further described below in conjunction with the accompanying drawings and specific embodiments.
[0041] The present invention provides a robust QoS-QoE prediction and guarantee system based on deep learning in a software-defined network, such as figure 1 As shown, first of all, the system is based on the software-defined network environment. The network architecture is divided into application layer, control layer and network layer. The client and server of the video streaming application run in the network topology composed of OpenFlow switches.
[0042] Since the SDN controller can monitor the topology of the underlying network and the port data flow of each switch to obtain the required network flow data, and at the same time perform related operations on the application layer and network layer, so if figure 1 As shown, each module of the present invention is deployed in the SDN controller of the control layer....
PUM
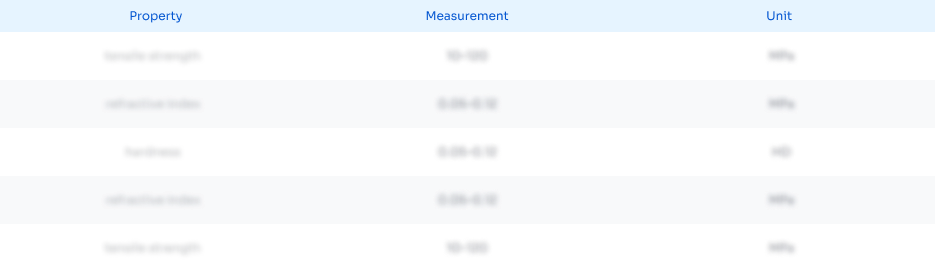
Abstract
Description
Claims
Application Information
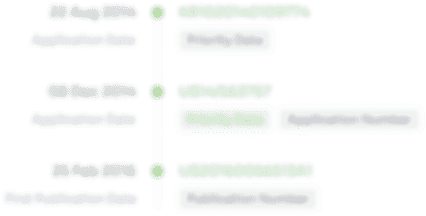
- R&D Engineer
- R&D Manager
- IP Professional
- Industry Leading Data Capabilities
- Powerful AI technology
- Patent DNA Extraction
Browse by: Latest US Patents, China's latest patents, Technical Efficacy Thesaurus, Application Domain, Technology Topic, Popular Technical Reports.
© 2024 PatSnap. All rights reserved.Legal|Privacy policy|Modern Slavery Act Transparency Statement|Sitemap|About US| Contact US: help@patsnap.com