Skin injury picture segmentation method based on a deep network
A skin damage and image segmentation technology, applied in the field of skin damage image segmentation based on deep network, can solve the problems of low running time, large changes in illumination and contrast, etc., achieve simple preprocessing, ensure integrity, and enrich the effect of training data
- Summary
- Abstract
- Description
- Claims
- Application Information
AI Technical Summary
Problems solved by technology
Method used
Image
Examples
Embodiment 1
[0043] like figure 1 As shown, a deep network-based skin lesion image segmentation method includes the following steps:
[0044] A method for segmenting skin damage pictures based on a deep network, comprising the following steps:
[0045] Step S1: Enhance and preprocess the test image;
[0046] Step S2: Input the preprocessed test image into the convolutional neural network for training, obtain preliminary segmentation results and probability outputs, and adjust the parameters of the convolutional neural network according to the preliminary segmentation results and probability outputs;
[0047] Step S3: Enhance and preprocess the training image;
[0048] Step S4: Input the preprocessed training image into the trained convolutional neural network for training, and obtain preliminary segmentation results and probability outputs;
[0049] Step S5: The segmentation result and probability output are iteratively processed in the fully connected conditional random field to obtain...
Embodiment 2
[0070] In this embodiment, the present invention is compared with the existing TDLS and Jafari methods for segmentation results and model running speed.
[0071] For the sake of fairness of comparison, the same experimental environment was set up in this embodiment, and 126 pictures from the DermQuest database were used as training data in the training phase of the model, including 66 melanoma pictures and 60 non-melanoma pictures. Due to the limited data, a cross-validation experimental plan was adopted, and the training data was randomly divided into 4 parts of equal size, and then 3 parts were selected for model training in turn, and the remaining 1 part was used as the evaluation set, and finally 4 parts were selected. The average of the experimental results. In terms of evaluation indicators, three indicators are used: true positive rate, true negative rate and accuracy rate.
[0072] Before the comparison, experiments are carried out to verify the necessity of the data ...
PUM
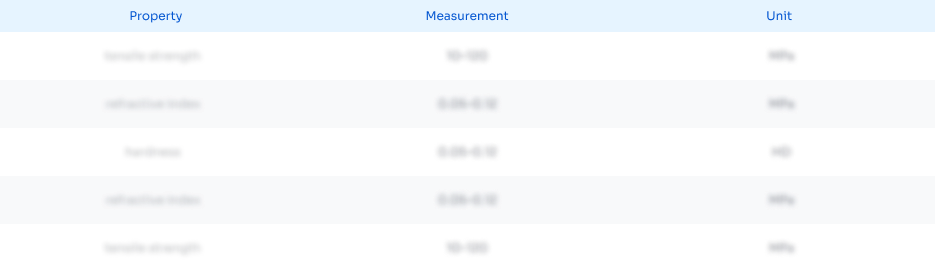
Abstract
Description
Claims
Application Information
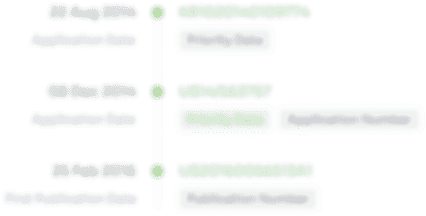
- R&D Engineer
- R&D Manager
- IP Professional
- Industry Leading Data Capabilities
- Powerful AI technology
- Patent DNA Extraction
Browse by: Latest US Patents, China's latest patents, Technical Efficacy Thesaurus, Application Domain, Technology Topic, Popular Technical Reports.
© 2024 PatSnap. All rights reserved.Legal|Privacy policy|Modern Slavery Act Transparency Statement|Sitemap|About US| Contact US: help@patsnap.com