An aspect-level emotion classification model and method based on dual-memory attention
An emotion classification and attention technology, applied in biological neural network models, semantic analysis, special data processing applications, etc., can solve the problem of ignoring emotional characteristics
- Summary
- Abstract
- Description
- Claims
- Application Information
AI Technical Summary
Problems solved by technology
Method used
Image
Examples
Embodiment Construction
[0070] The present invention will be further described below in conjunction with the accompanying drawings and embodiments.
[0071] This embodiment provides a RNN encoder-decoder sentiment classification model with dual memory attention, which consists of an encoder, two memory modules, a decoder and a classifier. First, the encoder encodes the word vector corresponding to the input sentence to obtain the hidden layer state in the GRU recurrent neural network and the intermediate vector And constitute two memory modules om and em, which respectively store potential word-level and phrase-level features; secondly, the decoder first performs the first decoding stage on em, and then performs the second decoding stage on om, which The aim is to capture phrase-level and word-level features from the two memories, respectively. In particular, the present invention adopts a special feed-forward neural network attention layer, continuously captures the important emotional features ...
PUM
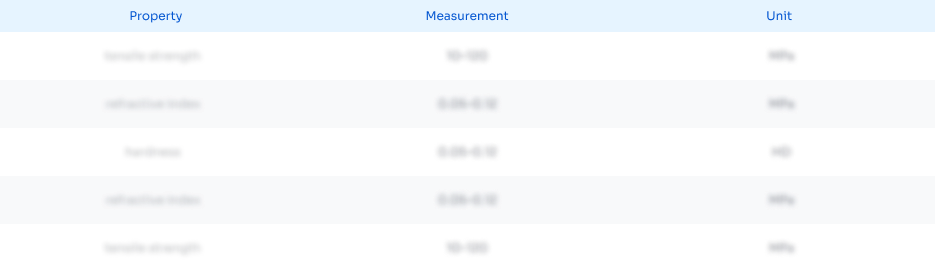
Abstract
Description
Claims
Application Information
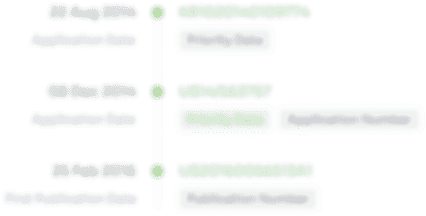
- R&D Engineer
- R&D Manager
- IP Professional
- Industry Leading Data Capabilities
- Powerful AI technology
- Patent DNA Extraction
Browse by: Latest US Patents, China's latest patents, Technical Efficacy Thesaurus, Application Domain, Technology Topic, Popular Technical Reports.
© 2024 PatSnap. All rights reserved.Legal|Privacy policy|Modern Slavery Act Transparency Statement|Sitemap|About US| Contact US: help@patsnap.com