Interactive method and system for semi-automatic image annotation
An interactive method and semi-automatic technology, applied in the field of interactive methods and systems for semi-automatic image labeling, can solve problems such as spending a lot of time sorting, under-fitting, and unsuitable sample labeling, so as to improve the recall rate and precision rate, improve The effect of generalization ability and saving labeling cost
- Summary
- Abstract
- Description
- Claims
- Application Information
AI Technical Summary
Problems solved by technology
Method used
Image
Examples
Embodiment Construction
[0046] An interactive method for semi-automatic labeling of images, comprising the steps of:
[0047] S1. Divide the initial sample into three different types of labeled samples according to different category attributes, namely subset 1, subset 2 and subset 3; output the above three types of labeled samples through manual labeling to obtain different types of labeling results, and then Use the three deep learning models of Mask-RCNN, Fast-RCNN, and FCN to train the above three subsets respectively, and finally obtain three classification models with different image region annotations;
[0048] According to the type of road features in the process of unmanned driving, the labeling category that needs to be labeled is designed, and a unique label is assigned to each category attribute. Since the gradient, texture and color characteristics of each category image will be different, the design Considerations required for each category during labeling.
[0049] When the number of ...
PUM
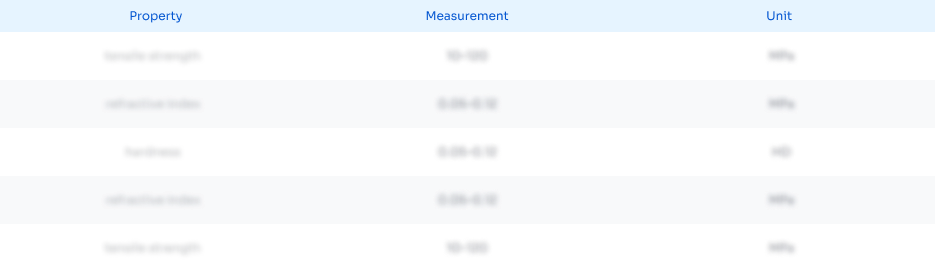
Abstract
Description
Claims
Application Information
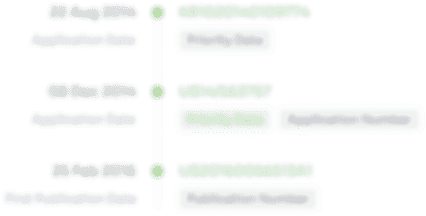
- R&D Engineer
- R&D Manager
- IP Professional
- Industry Leading Data Capabilities
- Powerful AI technology
- Patent DNA Extraction
Browse by: Latest US Patents, China's latest patents, Technical Efficacy Thesaurus, Application Domain, Technology Topic, Popular Technical Reports.
© 2024 PatSnap. All rights reserved.Legal|Privacy policy|Modern Slavery Act Transparency Statement|Sitemap|About US| Contact US: help@patsnap.com