Efficient quality inspection model construction method and system for self-learning and updating training samples
A technology of training samples and construction methods, applied in neural learning methods, biological neural network models, neural architectures, etc., can solve problems such as long prediction time, prevent the generation of local optimal models, ensure efficiency, and improve recognition efficiency. Effect
- Summary
- Abstract
- Description
- Claims
- Application Information
AI Technical Summary
Problems solved by technology
Method used
Image
Examples
Embodiment Construction
[0049] It should be noted that, in the case of no conflict, the embodiments of the present invention and the features in the embodiments can be combined with each other.
[0050] Content implementation of the present invention is described as follows:
[0051] 1. The symbols involved in the algorithm are expressed as follows:
[0052] L: Labeled sample set;
[0053] U: no sample label;
[0054] Z k : proxy label of the kth sample set;
[0055] m 0 : parent model, W 0 : the weight of the network structure of the parent model;
[0056] m i : the i-th training sub-model, i=1,2...n; let n be 2 in this article, that is, there is a sub-model M 1 and M 2 ;
[0057] W i : the weight of the sub-model network structure;
[0058] g 1 (x) and g 2 (x): Two image enhancement functions.
[0059] Loss: loss function.
[0060] Second, the algorithm is explained in detail as follows:
[0061] first part:
[0062] 1) That is: first use the label data L to train the SqueezeNet...
PUM
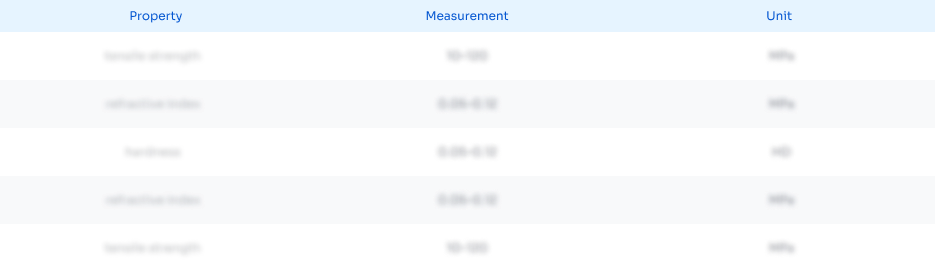
Abstract
Description
Claims
Application Information
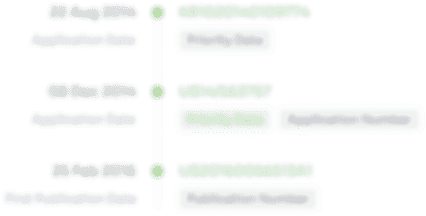
- R&D Engineer
- R&D Manager
- IP Professional
- Industry Leading Data Capabilities
- Powerful AI technology
- Patent DNA Extraction
Browse by: Latest US Patents, China's latest patents, Technical Efficacy Thesaurus, Application Domain, Technology Topic, Popular Technical Reports.
© 2024 PatSnap. All rights reserved.Legal|Privacy policy|Modern Slavery Act Transparency Statement|Sitemap|About US| Contact US: help@patsnap.com