A video gesture classification method method
A classification method and video technology, applied in the field of deep learning application research, can solve problems such as low recognition rate, and achieve an effect that is conducive to application promotion
- Summary
- Abstract
- Description
- Claims
- Application Information
AI Technical Summary
Problems solved by technology
Method used
Image
Examples
Embodiment
[0030] The processing of this embodiment is performed on the Chalearn LAP IsoGD dataset. This dataset was proposed by Wan et al. in the paper "Chalearn looking at people rgb-d isolated and continuous datasets for gesture recognition" on CVPRW2016. The dataset has a total of 47,933 gesture videos, and each video is made by a volunteer. Actions, the data set contains a total of 249 gestures. Among them, 35878 videos are used as training set, 5784 videos are used as verification set, and 6271 videos are used as test set.
[0031] refer to figure 1 , the video gesture classification method of this embodiment includes:
[0032] Step 1, read video data
[0033] Use matlab software to read video data, where the data includes visible light video (RGB video) and depth video (depth video) acquired by sensors such as Kinect.
[0034] Step 2, spatio-temporal normalization of video data
[0035] 2a) Spatial normalization of video data. The present invention normalizes the video in ste...
PUM
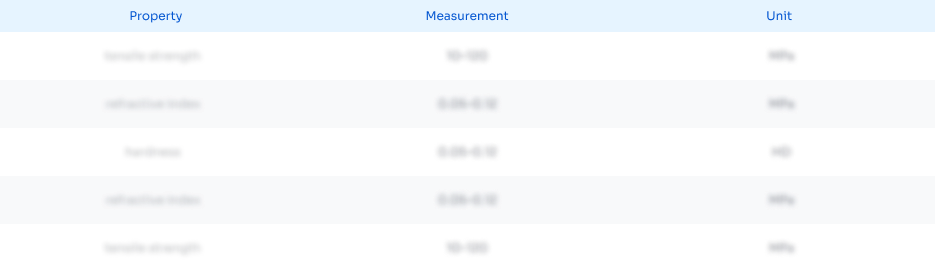
Abstract
Description
Claims
Application Information
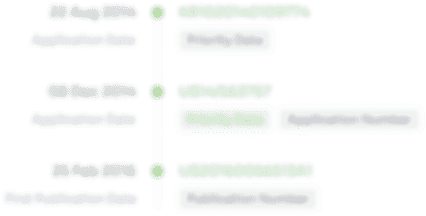
- R&D Engineer
- R&D Manager
- IP Professional
- Industry Leading Data Capabilities
- Powerful AI technology
- Patent DNA Extraction
Browse by: Latest US Patents, China's latest patents, Technical Efficacy Thesaurus, Application Domain, Technology Topic, Popular Technical Reports.
© 2024 PatSnap. All rights reserved.Legal|Privacy policy|Modern Slavery Act Transparency Statement|Sitemap|About US| Contact US: help@patsnap.com