A glaucoma medical image classification method based on capsule theory
A medical image and classification method technology, applied in the field of glaucoma medical image analysis, can solve the problem of low accuracy of glaucoma classification, achieve good training stability, fast convergence, and excellent accuracy
- Summary
- Abstract
- Description
- Claims
- Application Information
AI Technical Summary
Problems solved by technology
Method used
Image
Examples
Embodiment 1
[0042] A glaucoma medical image classification method based on capsule theory, comprising the following steps:
[0043] S1: Preprocessing the image;
[0044] S2: Construct a capsule-based convolutional neural network and input the preprocessed image, and output the glaucoma recognition result;
[0045] S3: Build an image reconstruction fully connected network, and use the category capsule to restore the original image.
[0046] In this embodiment, step S1 includes the following steps:
[0047] S1.1: Use the existing glaucoma medical imaging optic disc detection model to cut out the region of interest to reduce interference information;
[0048] S1.2: Fusion of optic disc and optic cup semantic segmentation map information;
[0049]S1.3: Adaptive histogram enhancement with limited contrast is used to improve the overall or local contrast of the image.
[0050] In this embodiment, the capsule-based convolutional neural network described in step S2 includes the first layer of...
Embodiment 2
[0067] Given a fundus map and its optic disc and cup segmentation map annotation information, the image preprocessing technology is used to process it as the input of the trained semantic segmentation network, and the classification probability is output after calculation to determine whether the original image is glaucoma. Process such as Figure 5 shown.
[0068] S1: Read the annotation information of the fundus map and its optic disc and cup segmentation map;
[0069] S2: Fusion of the fundus image and its segmentation image, the original 3-channel RGB fundus image and the single-channel segmentation image are superimposed into a new 4-channel image;
[0070] S3: Carry out the cropping operation of the optic disc area on the new 4-channel image to obtain a region of interest with an image size of 4×28×28;
[0071] S4: Use CLAHE to process the region of interest to enhance image or local contrast;
[0072] S5: use the preprocessed image as the input of the trained capsule...
PUM
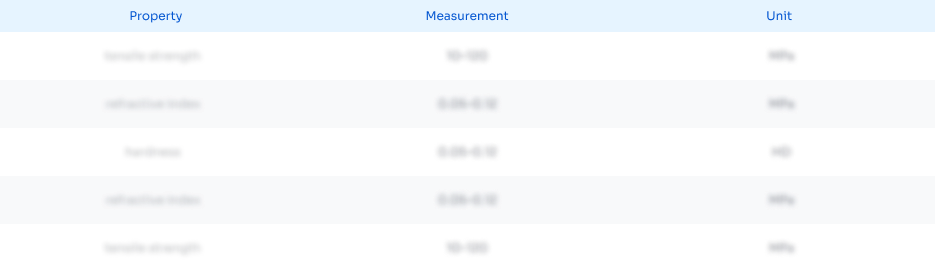
Abstract
Description
Claims
Application Information
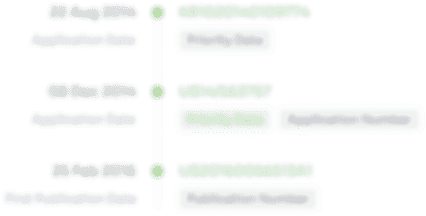
- R&D Engineer
- R&D Manager
- IP Professional
- Industry Leading Data Capabilities
- Powerful AI technology
- Patent DNA Extraction
Browse by: Latest US Patents, China's latest patents, Technical Efficacy Thesaurus, Application Domain, Technology Topic, Popular Technical Reports.
© 2024 PatSnap. All rights reserved.Legal|Privacy policy|Modern Slavery Act Transparency Statement|Sitemap|About US| Contact US: help@patsnap.com