Dual-energy CT image decomposition method based on CNN (convolutional neural network)
A convolutional neural network, CT image technology, applied in neural learning methods, biological neural network models, image analysis, etc., can solve problems such as large noise and low signal-to-noise ratio, and achieve the effect of improving training efficiency
- Summary
- Abstract
- Description
- Claims
- Application Information
AI Technical Summary
Problems solved by technology
Method used
Image
Examples
Embodiment 1
[0038] Such as figure 1 As shown, a kind of convolutional neural network-based dual-energy CT image decomposition method of the present invention comprises the following steps:
[0039] Step S101: Design a double-input, double-output convolutional neural network model as the mapping function D(μ H,L ; Θ), where μ H,L For a dual-energy CT image, the convolutional neural network model is designed as a network structure model with double input and double output, such as figure 2 As shown, A and B in the figure are the input high-energy CT images and low-energy CT images, M1 and M2 are the output base material 1 and base material 2, "→" represents two different network structures; and in the dual-input and dual-output Cross convolution is established in the network structure model, such as image 3 Shown; Short links are established in the convolutional neural network model. The specific convolutional neural network model is as follows Figure 4 Shown: Among them, the convol...
PUM
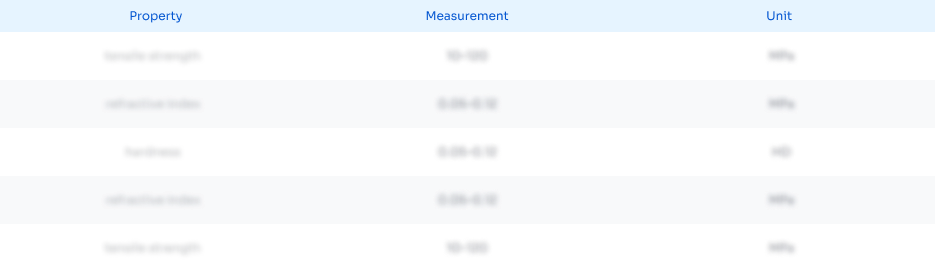
Abstract
Description
Claims
Application Information
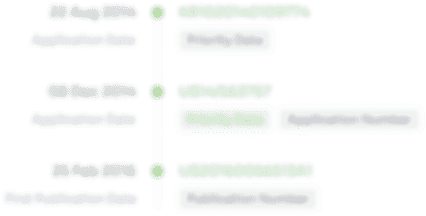
- R&D Engineer
- R&D Manager
- IP Professional
- Industry Leading Data Capabilities
- Powerful AI technology
- Patent DNA Extraction
Browse by: Latest US Patents, China's latest patents, Technical Efficacy Thesaurus, Application Domain, Technology Topic, Popular Technical Reports.
© 2024 PatSnap. All rights reserved.Legal|Privacy policy|Modern Slavery Act Transparency Statement|Sitemap|About US| Contact US: help@patsnap.com