Face age estimation method performing measurement learning based on convolutional neural network
A convolutional neural network and metric learning technology, applied in neural learning methods, biological neural network models, neural architectures, etc., can solve the problems that feature extraction cannot optimize function services, age distribution is not ideal, etc., to achieve accurate age, distinguishing High degree of robustness
- Summary
- Abstract
- Description
- Claims
- Application Information
AI Technical Summary
Problems solved by technology
Method used
Image
Examples
Embodiment Construction
[0025] The present invention will be described in further detail below with reference to the accompanying drawings and specific embodiments.
[0026] A face age estimation method based on metric learning based on convolutional neural network, the overall steps are:
[0027] Step 1. Data extraction stage: use the existing face detection engine to perform face detection and 5-point (2 eye corners, nose tip, 2 mouth corners) positioning on the face RGB image, and align the face image according to the 5-point position , remove the in-plane rotation change and normalize the face size, and finally cut out the face area and save the face image as 256×256 pixels. This step includes but is not limited to face alignment based on 5 points.
[0028] Step 2. Age data set division: In order to ensure the generalization ability of the model and avoid overfitting on the training set, the data set needs to be divided. The age data set is randomly divided according to 80% as the training set ...
PUM
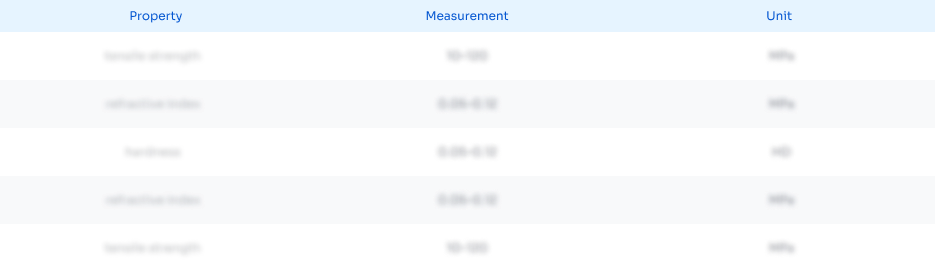
Abstract
Description
Claims
Application Information
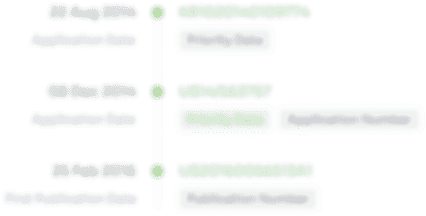
- R&D Engineer
- R&D Manager
- IP Professional
- Industry Leading Data Capabilities
- Powerful AI technology
- Patent DNA Extraction
Browse by: Latest US Patents, China's latest patents, Technical Efficacy Thesaurus, Application Domain, Technology Topic, Popular Technical Reports.
© 2024 PatSnap. All rights reserved.Legal|Privacy policy|Modern Slavery Act Transparency Statement|Sitemap|About US| Contact US: help@patsnap.com