Deep-belief-network-based diagnosis method of distribution network
A deep belief network and distribution network fault technology, applied in the field of distribution network, can solve the problems of poor fault analysis effect, cumbersome calculation and large diagnosis error, etc.
- Summary
- Abstract
- Description
- Claims
- Application Information
AI Technical Summary
Problems solved by technology
Method used
Image
Examples
Embodiment Construction
[0024] In order to facilitate those of ordinary skill in the art to understand and implement the present invention, the present invention will be described in further detail below in conjunction with the accompanying drawings and embodiments. It should be understood that the implementation examples described here are only used to illustrate and explain the present invention, and are not intended to limit this invention.
[0025] please see figure 1 , the present invention is based on the distribution network fault diagnosis method of depth belief network, comprises the following steps:
[0026] Step 1: Obtain the data collected by the feeder terminal unit (FTU) and other equipment at the time of the distribution network fault, including switch status, power parameters, phase-to-phase fault, ground fault, and fault parameters;
[0027] Step 2: Preprocess the original data, remove redundant data and "bad data" in the original data, and normalize the collected data of different ...
PUM
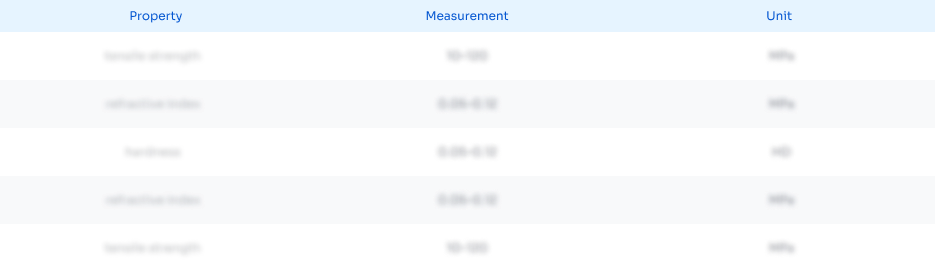
Abstract
Description
Claims
Application Information
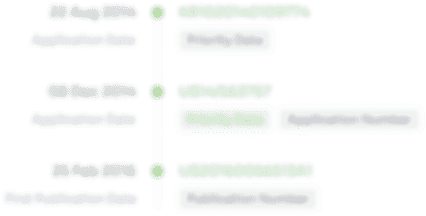
- Generate Ideas
- Intellectual Property
- Life Sciences
- Materials
- Tech Scout
- Unparalleled Data Quality
- Higher Quality Content
- 60% Fewer Hallucinations
Browse by: Latest US Patents, China's latest patents, Technical Efficacy Thesaurus, Application Domain, Technology Topic, Popular Technical Reports.
© 2025 PatSnap. All rights reserved.Legal|Privacy policy|Modern Slavery Act Transparency Statement|Sitemap|About US| Contact US: help@patsnap.com