Channel logo segmentation method based on fully convolutional channel logo segmentation network
A technology of station logo and convolution, applied in neural learning methods, biological neural network models, image analysis, etc., can solve the problems of large background influence, uneven video images, multiple background noises, etc., to achieve strong description ability and discrimination ability, Strong feature description ability and the effect of improving spatial accuracy
- Summary
- Abstract
- Description
- Claims
- Application Information
AI Technical Summary
Problems solved by technology
Method used
Image
Examples
Embodiment Construction
[0029] A logo segmentation method based on a fully convolutional logo segmentation network of the present invention will be described in detail below with reference to the embodiments and the accompanying drawings.
[0030] A logo segmentation method based on a fully convolutional logo segmentation network of the present invention, firstly, extracts representative frames from the video sample library, and obtains multiple types of The fine-grained station logo image set; label the pixels in the station logo image set one by one, and obtain the corresponding binary label image set. Next, a fully convolutional logo segmentation network (Logo-SegNet) with an encoder-decoder structure is constructed, and a deep convolutional feature training network is extracted from the logo image set. Finally, the trained network is used to segment the multi-type fine-grained logo image sets.
[0031] Such as figure 1 As shown, a kind of station logo segmentation method based on the full convo...
PUM
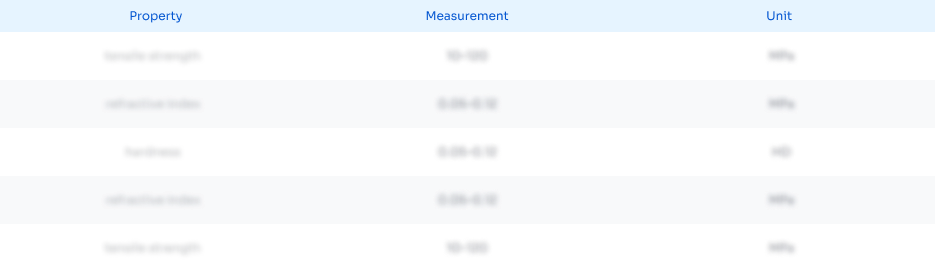
Abstract
Description
Claims
Application Information
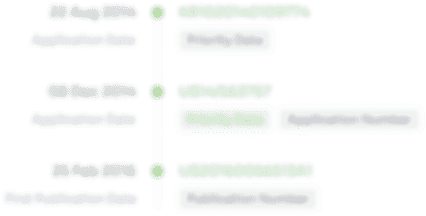
- R&D Engineer
- R&D Manager
- IP Professional
- Industry Leading Data Capabilities
- Powerful AI technology
- Patent DNA Extraction
Browse by: Latest US Patents, China's latest patents, Technical Efficacy Thesaurus, Application Domain, Technology Topic, Popular Technical Reports.
© 2024 PatSnap. All rights reserved.Legal|Privacy policy|Modern Slavery Act Transparency Statement|Sitemap|About US| Contact US: help@patsnap.com