General miniaturization method of deep neural network
A deep neural network and small-scale network technology, applied in biological neural network models, neural learning methods, neural architectures, etc., can solve problems such as performance degradation, achieve good stability and effectiveness, reduce network calculations, and reduce storage effect of space
- Summary
- Abstract
- Description
- Claims
- Application Information
AI Technical Summary
Problems solved by technology
Method used
Image
Examples
Embodiment Construction
[0019] In order to further understand the features, technical means, and specific objectives and functions achieved by the present invention, the present invention will be further described in detail below in conjunction with the accompanying drawings and specific embodiments.
[0020] The present invention discloses a general miniaturization method of a deep neural network, which can be widely applied to deep neural network models deployed on embedded and mobile computing platforms, specifically comprising the following steps:
[0021] Perform feature reconstruction on the initial deep neural network to form a new small network. Reconstruct the initial large and complex network, and use the reconstruction method to compress the deep neural network, so that the original deep neural network can be effectively simplified.
[0022] The number of feature channels and the number of group convolutions of the deep neural network are compressed to reduce the number of output feature c...
PUM
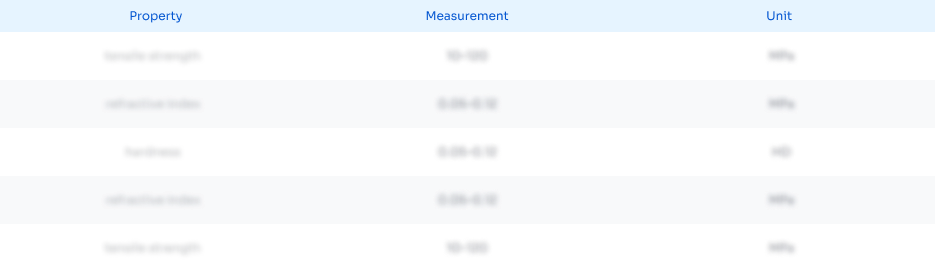
Abstract
Description
Claims
Application Information
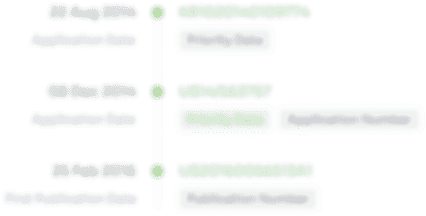
- R&D Engineer
- R&D Manager
- IP Professional
- Industry Leading Data Capabilities
- Powerful AI technology
- Patent DNA Extraction
Browse by: Latest US Patents, China's latest patents, Technical Efficacy Thesaurus, Application Domain, Technology Topic, Popular Technical Reports.
© 2024 PatSnap. All rights reserved.Legal|Privacy policy|Modern Slavery Act Transparency Statement|Sitemap|About US| Contact US: help@patsnap.com