CT image pulmonary nodule detection method based on 3D residual neural network
A technology of CT image and detection method, which is applied in medical image analysis, machine learning, and medical image analysis based on deep learning. It can solve the problems of low accuracy and achieve the effects of saving costs, reducing feature omissions, and increasing complexity.
- Summary
- Abstract
- Description
- Claims
- Application Information
AI Technical Summary
Problems solved by technology
Method used
Image
Examples
example
[0087] Example: There are two types of CT images of pulmonary nodules used in this case, including pulmonary nodules and healthy. There are a total of 1200 samples of pulmonary nodules. The number of healthy samples is the same as that of lung nodules. The healthy samples have all tissue samples in the chest cavity to ensure the diversity of negative samples. Select 100 samples from the positive and negative samples as the verification set, 100 samples as the test set, and the remaining 1000 samples as the training set. The operation steps include model building, model training and model testing.
[0088] Step 1, construct a three-dimensional deep convolutional neural network, the specific structure is as follows figure 2 shown.
[0089] Step 1.1: This convolutional neural network consists of 5 convolutional modules, 4 AveragePooling layers, 4 fusion layers and 1 Dense Branch module.
[0090] Step 1.2: In the convolution layer, the size of the convolution kernel is 3*3*3, ...
PUM
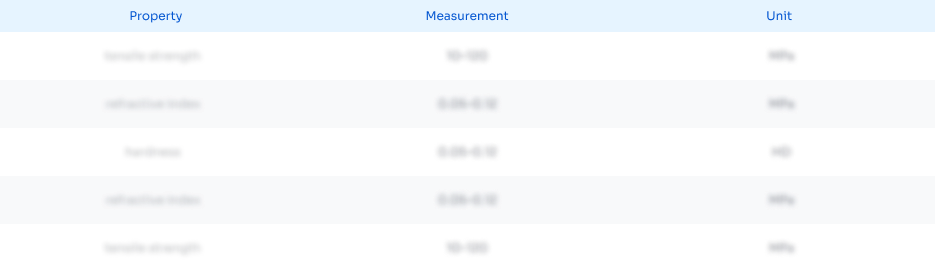
Abstract
Description
Claims
Application Information
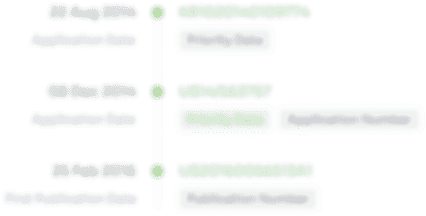
- Generate Ideas
- Intellectual Property
- Life Sciences
- Materials
- Tech Scout
- Unparalleled Data Quality
- Higher Quality Content
- 60% Fewer Hallucinations
Browse by: Latest US Patents, China's latest patents, Technical Efficacy Thesaurus, Application Domain, Technology Topic, Popular Technical Reports.
© 2025 PatSnap. All rights reserved.Legal|Privacy policy|Modern Slavery Act Transparency Statement|Sitemap|About US| Contact US: help@patsnap.com