Bayesian network platform with self-learning function
A Bayesian network and self-learning technology, applied in the field of engineering applications, can solve problems such as unsatisfactory, and achieve the effects of strong adaptability, convenient and flexible operation, and high computing efficiency
- Summary
- Abstract
- Description
- Claims
- Application Information
AI Technical Summary
Problems solved by technology
Method used
Image
Examples
Embodiment 1
[0022] Among the various short circuits that occur in the three-phase AC lines of the power grid, the single-phase ground short circuit accounts for the highest proportion, about 65%, the two-phase ground short circuit accounts for about 20%, the two-phase short circuit accounts for about 10%, and the three-phase short circuit accounts for the smallest. About 5%. In the single-phase grounding short-circuit fault, the three reasons of wire disconnection, insulator breakdown and tree short-circuit account for 80% of the total fault reasons, which are about 30%, 30% and 20% respectively, and other reasons only account for 20%.
[0023] figure 1 for the network structure. Call the sample data set in Table 1, execute the network structure learning module function, create a network structure, and then further define node attributes, including: name, title, type, discrete and continuous attributes, number of states, state values, and related descriptions, etc.
[0024] Table 1 Samp...
Embodiment 2
[0028] image 3 For predictive analysis, set the "Line breakdown" variable state to Present=100% in the Bayesian network, indicating the known state of the evidence variable, and the automatic update function automatically updates the probability of the entire network. At this time, the probability of single-phase ground short circuit (1-phase ground) appearing (Present) changed from 50.7% to 64.2%, and the probability of short circuit fault (Short circuit) appearing (Present) changed from 52.9% to 61.7%. It can be seen that the wire is broken After the line, the probability of short circuit failure is increased.
[0029] Figure 4 For wire breakage and insulator breakdown (Insulator breakdown) to occur at the same time, set the variable state of the evidence node "Insulator breakdown" to Present=100%, and use the automatic update function to update the probability of the entire network. At this time, the probability of single-phase ground short circuit (1-phase ground) appe...
Embodiment 3
[0033] Table 2, according to figure 2 The shown Bayesian network is used as the platform, and the sensitivity analysis of "short-circuit fault" is used as the query node.
[0034] Table 2 Evidence sensitivity analysis with "short circuit fault" as the query node
[0035]
[0036]
[0037] Each row in Table 2 indicates that if the node evidence in column 1 is obtained, then columns 3, 4, and 5 are the minimum, current, and maximum posterior probabilities in the case of Short-C=Present; column 6 and column 7 are the absolute value and percentage of entropy reduction; column 8 is the variance.
[0038] It can be seen from Table 2 that the nodes that have influence on short-circuit faults are their parent nodes and child nodes. Also, the evidence most likely to have the greatest impact on short-circuit fault reliability is listed first.
PUM
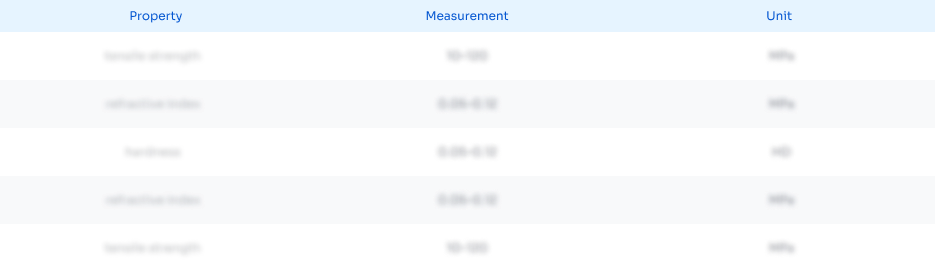
Abstract
Description
Claims
Application Information
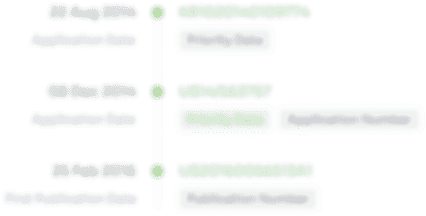
- R&D Engineer
- R&D Manager
- IP Professional
- Industry Leading Data Capabilities
- Powerful AI technology
- Patent DNA Extraction
Browse by: Latest US Patents, China's latest patents, Technical Efficacy Thesaurus, Application Domain, Technology Topic, Popular Technical Reports.
© 2024 PatSnap. All rights reserved.Legal|Privacy policy|Modern Slavery Act Transparency Statement|Sitemap|About US| Contact US: help@patsnap.com