Multi-example learning method using deep learning technology
A multi-instance learning and deep learning technology, applied in neural learning methods, instruments, biological neural network models, etc., can solve problems such as poor algorithm robustness, classification model accuracy and generalization performance limitations, and training dataset information loss.
- Summary
- Abstract
- Description
- Claims
- Application Information
AI Technical Summary
Problems solved by technology
Method used
Image
Examples
Embodiment Construction
[0038] The present invention is tested on the UCI data set (http: / / archive.ics.uci.edu / ml / ) and has achieved good results. An example is given below, taking the Musk2( http: / / archive.ics.uci.edu / ml / datasets / Musk+%28Version+2%29 ) As a test data set, this data set is a multi-example data set, with 6598 examples and 168 data attributes, all of which are continuous attributes. The minimum number of examples contained in the sample is 13, and the maximum number of examples contained in the sample is 51 .
[0039] (1) Data preprocessing
[0040] For continuous attributes, find the maximum and minimum values of the attributes in the data set, and use the preprocessing method for continuous attributes of the present invention to process them. For example: for a continuous field f1, find its maximum and minimum values in all data, respectively, 292 and -3, then for the field of the first record in the data set, its normalized value is: (46-(-3)) / (292-(-3))=0.661. In this embodime...
PUM
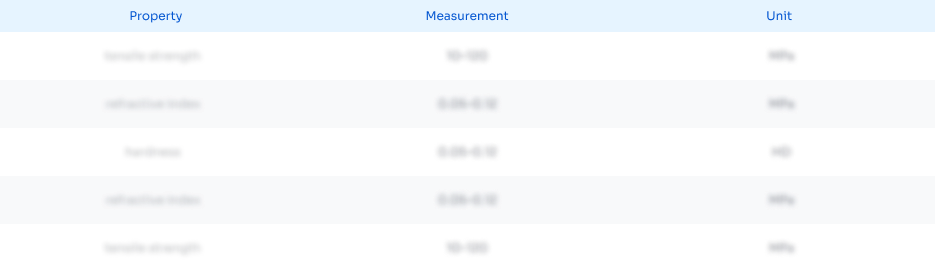
Abstract
Description
Claims
Application Information
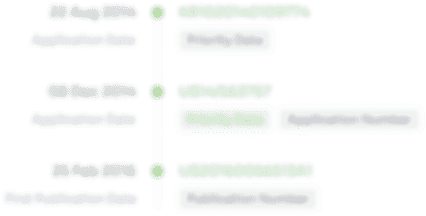
- R&D Engineer
- R&D Manager
- IP Professional
- Industry Leading Data Capabilities
- Powerful AI technology
- Patent DNA Extraction
Browse by: Latest US Patents, China's latest patents, Technical Efficacy Thesaurus, Application Domain, Technology Topic, Popular Technical Reports.
© 2024 PatSnap. All rights reserved.Legal|Privacy policy|Modern Slavery Act Transparency Statement|Sitemap|About US| Contact US: help@patsnap.com