Neural network increment-type feedforward algorithm based on sample increment driving
A neural network and incremental technology, applied in the algorithm field of online learning single hidden layer feedforward neural network, can solve the problems of poor prediction effect and stability of neural network, improve prediction accuracy and generalization ability, and satisfy dynamic optimization The effect of controlling requirements and improving stability
- Summary
- Abstract
- Description
- Claims
- Application Information
AI Technical Summary
Problems solved by technology
Method used
Image
Examples
Embodiment Construction
[0022] The present invention will be further described below in conjunction with accompanying drawing:
[0023] Such as figure 1 As shown, the algorithm steps of the present invention are as follows:
[0024] Step 1. In the neural network, select any time L 0 training samples Initialize the model parameters; randomly set an m×n matrix P, m is the number of hidden layer nodes, and n is the number of input nodes. Compute Composite Matrix
[0025] Step 2, calculate the input weight and hidden layer threshold;
[0026] Step 3, and then calculate the hidden layer output matrix H 0 ;
[0027] Step 4. Calculate the output weight matrix β according to the least squares method and MP generalized inverse method 0 ; Set the parameter k=0, where k is the sequence number of samples added.
[0028] Step 5, introduce a new sample X 1 , calculate the L-th difference between the new sample and the initial training sample 0 Whether there is an increment between samples, if there is...
PUM
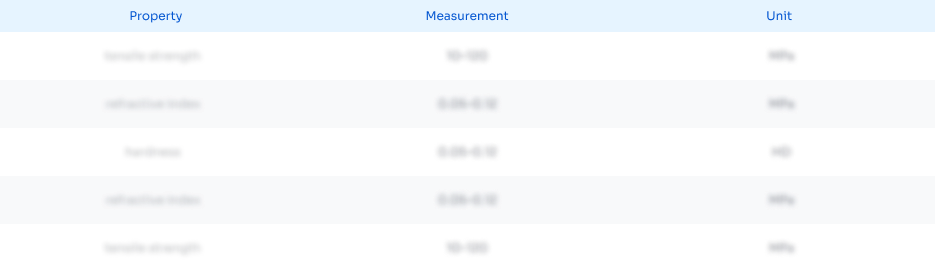
Abstract
Description
Claims
Application Information
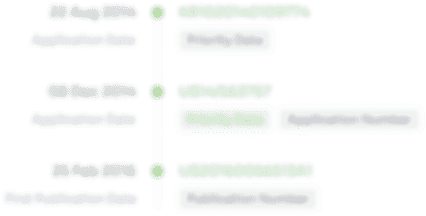
- Generate Ideas
- Intellectual Property
- Life Sciences
- Materials
- Tech Scout
- Unparalleled Data Quality
- Higher Quality Content
- 60% Fewer Hallucinations
Browse by: Latest US Patents, China's latest patents, Technical Efficacy Thesaurus, Application Domain, Technology Topic, Popular Technical Reports.
© 2025 PatSnap. All rights reserved.Legal|Privacy policy|Modern Slavery Act Transparency Statement|Sitemap|About US| Contact US: help@patsnap.com