An Image Annotation Method Based on Weak Matching Probability Canonical Correlation Model
A canonical correlation and matching probability technology, which is applied in the field of image annotation based on the weak matching probability canonical correlation model, which can solve the problems of time-consuming and laborious manual matching, data asynchrony, and missing data.
- Summary
- Abstract
- Description
- Claims
- Application Information
AI Technical Summary
Problems solved by technology
Method used
Image
Examples
specific Embodiment
[0100] The following are specific embodiments of the present invention, as follows:
[0101] Experiments on artificial weak matching multimodal datasets are as follows:
[0102] In order to verify the effectiveness of the SemiPCCA model, we construct the following artificial data sets: sample set subject to N(0,I d ), where the dimension d=2, the number of samples N=300, the complete set of matching samples Obtained by constructing,
[0103]
[0104]
[0105]
[0106] In order to obtain a weakly matched sample set, we construct a discriminant function f(x 2 ) = a T x 2 -θ, where θ represents the discrimination threshold, for the sample If its discriminant function value then from remove the sample. It can be seen that the larger θ is, the more samples are removed;
[0107] When comparing SemiPCCA with traditional CCA and PCCA, we chose the following weighted cosine distance,
[0108]
[0109] in, and Respectively represent the "true" d typical p...
PUM
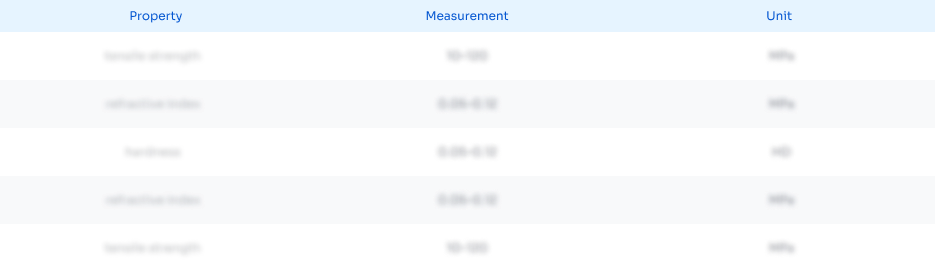
Abstract
Description
Claims
Application Information
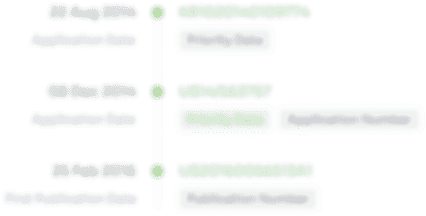
- R&D Engineer
- R&D Manager
- IP Professional
- Industry Leading Data Capabilities
- Powerful AI technology
- Patent DNA Extraction
Browse by: Latest US Patents, China's latest patents, Technical Efficacy Thesaurus, Application Domain, Technology Topic, Popular Technical Reports.
© 2024 PatSnap. All rights reserved.Legal|Privacy policy|Modern Slavery Act Transparency Statement|Sitemap|About US| Contact US: help@patsnap.com