Noise variance estimating method based on broad sense autoregression heteroscedasticity model
A noise variance estimation and autoregressive technology, applied in the field of image processing, to achieve the effect of improving accuracy
- Summary
- Abstract
- Description
- Claims
- Application Information
AI Technical Summary
Problems solved by technology
Method used
Image
Examples
specific Embodiment approach
[0062] A noise variance estimation method based on the generalized autoregressive heteroscedastic model of the present invention, such as Figure 1 to Figure 3 shown, including the following steps:
[0063] Step 1, read the noisy image g contaminated by noise x,y .
[0064] Read a noisy image contaminated by noise g x,y = f x,y +n x,y , where f x,y Indicates a clear image, n x,y Represents a noisy image, the noisy image g x,y , clear image f x,y , noise image n x,y The size is N×M, n x,y Obedience mean is 0, variance is σ 2 The normal distribution of n x,y ~N(0,σ 2 ), the subscripts x and y represent the row coordinates and column coordinates of the image respectively, 1≤x≤N, 1≤y≤M.
[0065] Step 2, for the noisy image g polluted by noise x,y Do a non-subsampled contourlet transform, i.e. NSCT.
[0066] For noise-contaminated images g x,y Do L-level non-sub-sampling contourlet transformation (the best value of L in this implementation example is 3 or 4), where ...
PUM
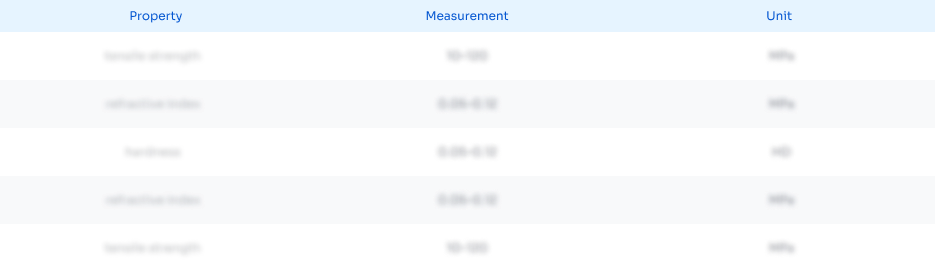
Abstract
Description
Claims
Application Information
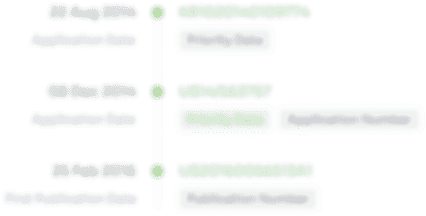
- R&D
- Intellectual Property
- Life Sciences
- Materials
- Tech Scout
- Unparalleled Data Quality
- Higher Quality Content
- 60% Fewer Hallucinations
Browse by: Latest US Patents, China's latest patents, Technical Efficacy Thesaurus, Application Domain, Technology Topic, Popular Technical Reports.
© 2025 PatSnap. All rights reserved.Legal|Privacy policy|Modern Slavery Act Transparency Statement|Sitemap|About US| Contact US: help@patsnap.com